In this article, we will delve into the architecture and operational mechanisms of the decoder in transformer models, which are integral to tasks such as language translation and text generation. We will outline the sequential processing of input matrices, starting from token embeddings scaled by the number of units, through the application of dropout for regularization, and the creation of future and memory masks. These steps ensure that the decoder can effectively manage context and dependencies within sequences.
We will also examine the series of transformations that occur within each layer of the decoder, including self-attention and cross-attention processes. This involves calculating queries, keys, and values, along with applying normalization and dropout at various stages. Finally, we will discuss how the outputs from the decoder are transformed into logits, ready for generating predictions, and highlight the call sequence of functions and classes that orchestrate these complex operations. Through this exploration, we aim to provide a comprehensive understanding of the decoder's role in transformer architectures.
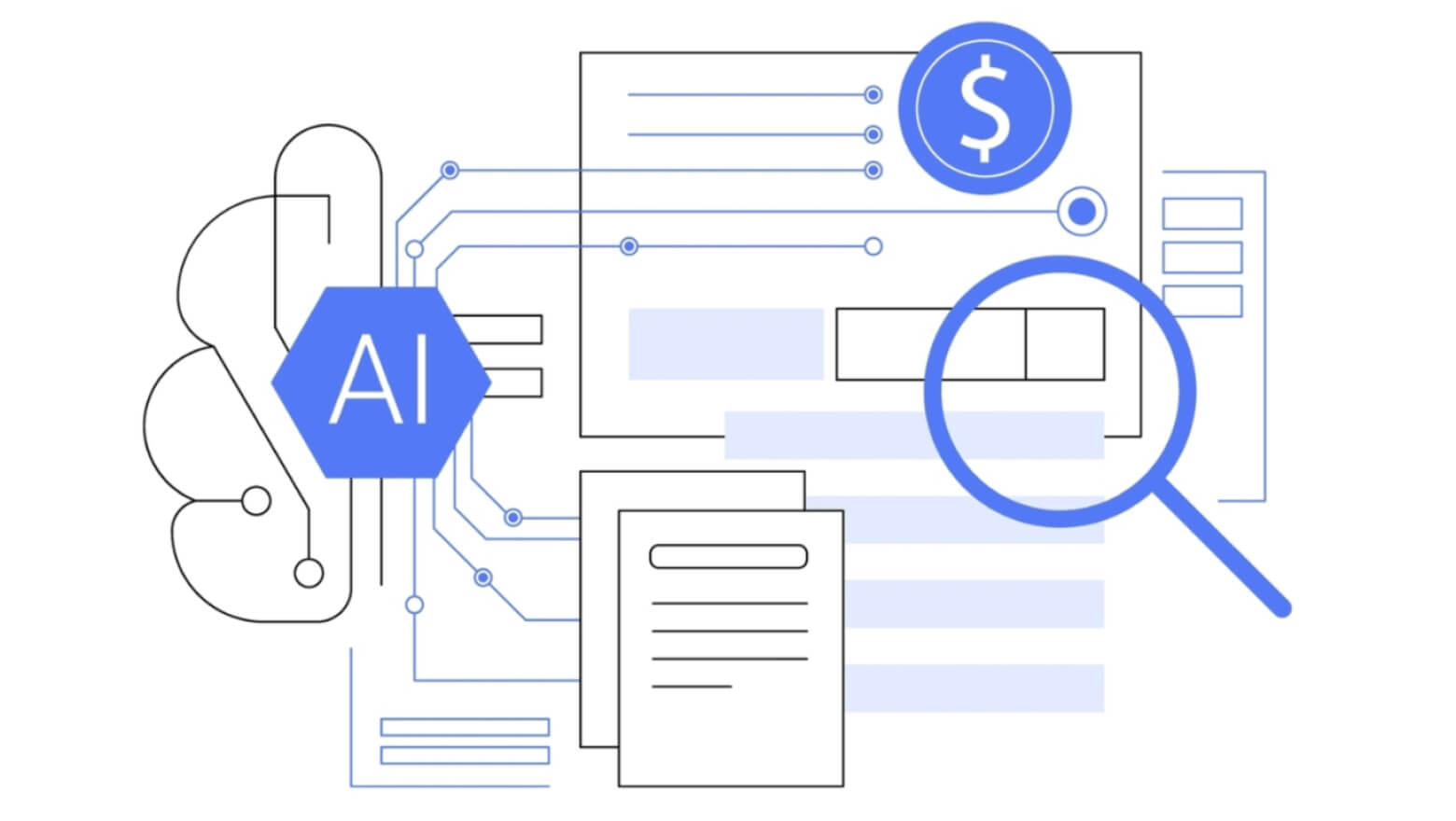
Decoder
In DECODER VECTOR PROPOSITION OF TOKENS target LANGUAGE:
The generated inputs matrix using the function tf.nn.embedding_lookup is multiplied by the square root of num_units (in our example num_units=4) → inputs = inputs * √numunits. (Image 1 - num_units)
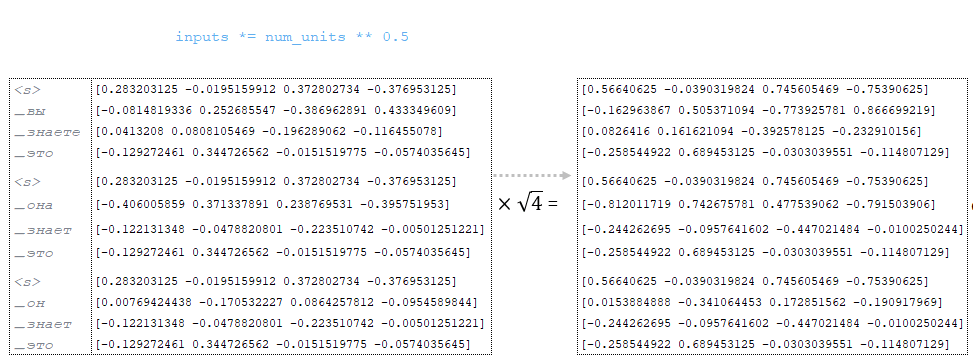
Dropout is applied (dropout parameter from the configuration file). (Image 2 - dropout )
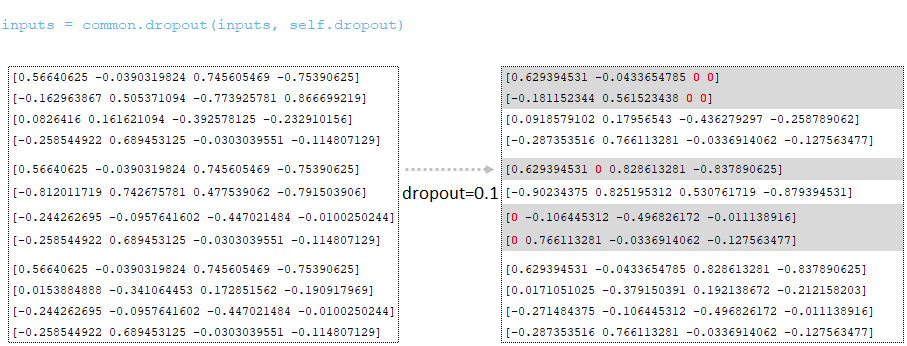
On the dimensionality of the inputs matrix batches, the future_mask tensor is built with the help of the future_mask function. When training the decoder, future tokens of the sequence will be hidden, the decoder has access only to the current token and previous tokens. (Image 3 - future_mask)
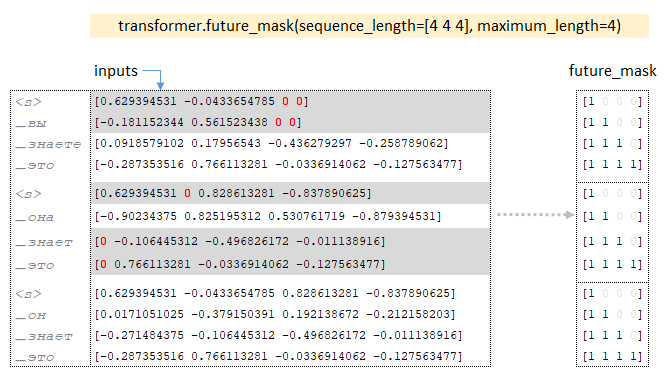
Memory_mask tensor is formed using the matrix obtained in the encoder using the tf.sequence_mask function. (Image 4 - memory_mask)
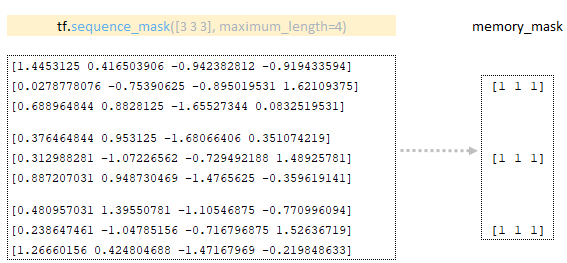
In the loop, for each layer, equal to the number of Layers parameter the inputs matrix, future_mask tensor, memory_mask tensor and the matrix obtained after encoder encoder_outputs are passed to the layer, the result returned by layer - inputs is passed to the next layer. For example, if we have 6 layers, the result from the first layer will be the input result for the second layer, etc. From each layer, the attention matrix formed in the cross_attention (or encoder-decoder attention) layer is returned and stored in a list. (Image 5 - attention matrix)

In each layer, the transformations described below take place. Inputs matrix and future_mask matrix are transformed using the self attention layer. The full mechanism of matrix transformations is described in the encoder section. Here we list the main steps:
━ 1) the inputs matrix is passed through the normalization layer;
━ 2) the matrix of queries is calculated and divided by the number of heads;
━ 3) the queries matrix divided by the number of heads is divided by the square root of num_units_per_head;
━ 4) the matrix keys is calculated and divided by the number of heads;
━ 5) the matrix values is calculated and divided by the number of heads;
━ 6) relative_repr_keys and relative_repr_values matrices are calculated;
━ 7) scalar product of the matrices queries and keys is obtained;
━ 8) the queries matrix is multiplied with the matrix relative_repr_key;
━ 9) matrix matmul_with_relative_representations is added to the scalar product of matrices;
━ 10) transformation with matrix future_mask;
━ 11) softmax activation function is applied;
━ 12) dropout is applied (parameter attention_dropout from configuration file);
━ 13) multiplication with the values matrix to form the matrix heads;
━ 14) multiplication with the matrix relative_repr_values;
━ 15) addition of the matrix heads with the matrix matmul_with_relative_representations;
━ 16) combining heads (matrix heads) into a common matrix;
━ 17) linear transformation;
━ 18) dropout applied (dropout parameter from configuration file);
━ 19) residual connection mechanism is applied.
After the above transformations we obtain the outputs matrix . (Image 6 - outputs matrix)
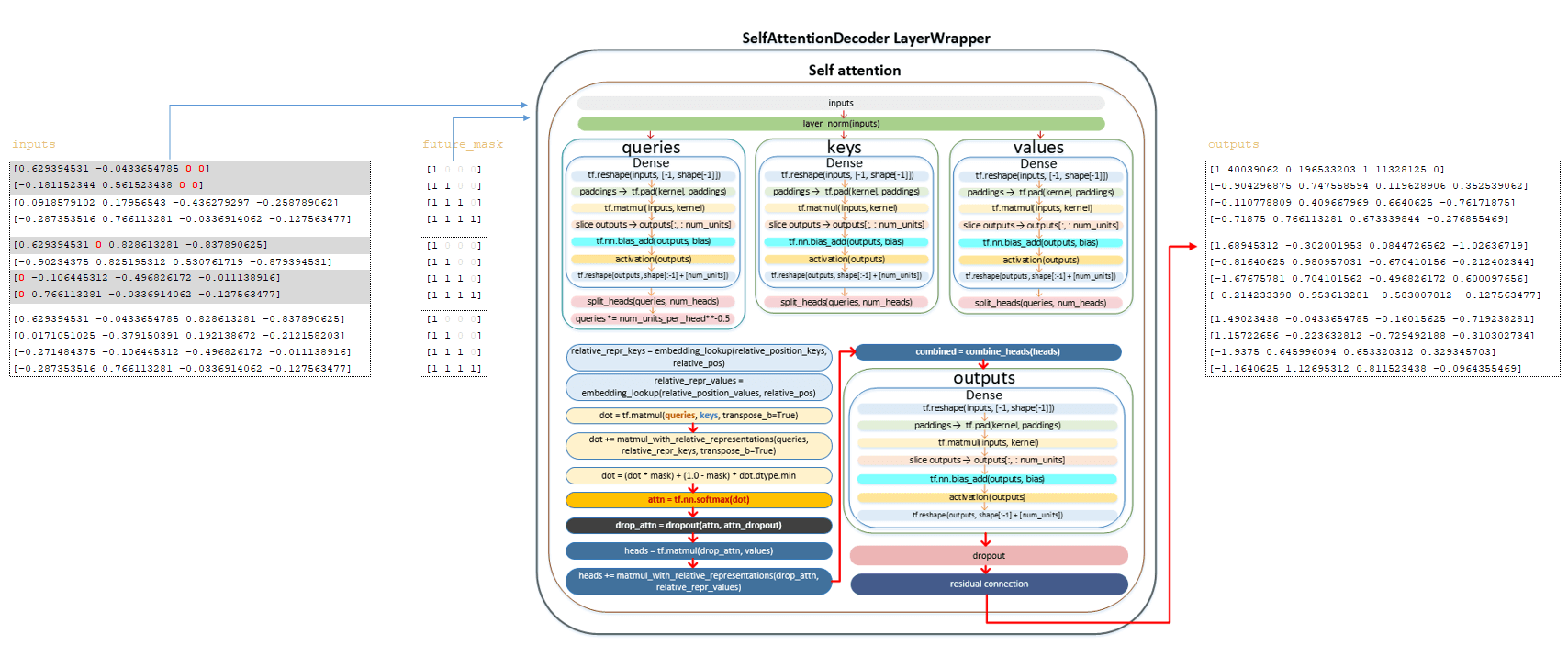
The matrix after the self attention layer, the memory_mask matrix, and the matrix obtained in encoder_outputs are fed to the input of the cross attention layer.
The full mechanism of matrix transformations is described in the encoder section. Here we will list the main steps:
━ 1) inputs matrix is passed through the normalization layer;
━ 2) the matrix of queries is calculated and divided by the number of heads;
━ 3) the queries matrix divided by the number of heads is divided by the square root of num_units_per_head ;
━ 4) the keys matrix is calculated from the encoder matrix and divided by the number of heads;
━ 5) the values matrix is calculated using the encoder matrix and divided by the number of heads;
━ 6) the matrix relative_repr_keys and relative_repr_values is calculated;
━ 7) scalar product of the matrices queries and keys is obtained;
━ 8) the queries matrix is multiplied with the matrix relative_repr_key ;
━ 9) matrix matmul_with_relative_representations is added to the scalar product of matrices;
━ 10) transformation with matrix memory_mask ;
━ 11) softmax activation function is applied, we get the attention matrix which is returned from this layer ;
━ 12) dropout is applied (parameter attention_dropout from the configuration file);
━ 13) multiplication with the values matrix to form the heads matrix;
━ 14) multiplication with the matrix relative_repr_values ;
━ 15) addition of the matrix heads with the matrix matmul_with_relative_representations ;
━ 16) combining heads (matrix heads ) into a common matrix;
━ 17) linear transformation;
━ 18) dropou t applied ( dropout parameter from configuration file);
━ 19) residual connection mechanism is applied.
After the above transformations we obtain the outputs matrix and the attention matrix. (Image 7 - outputs and attention matrix)
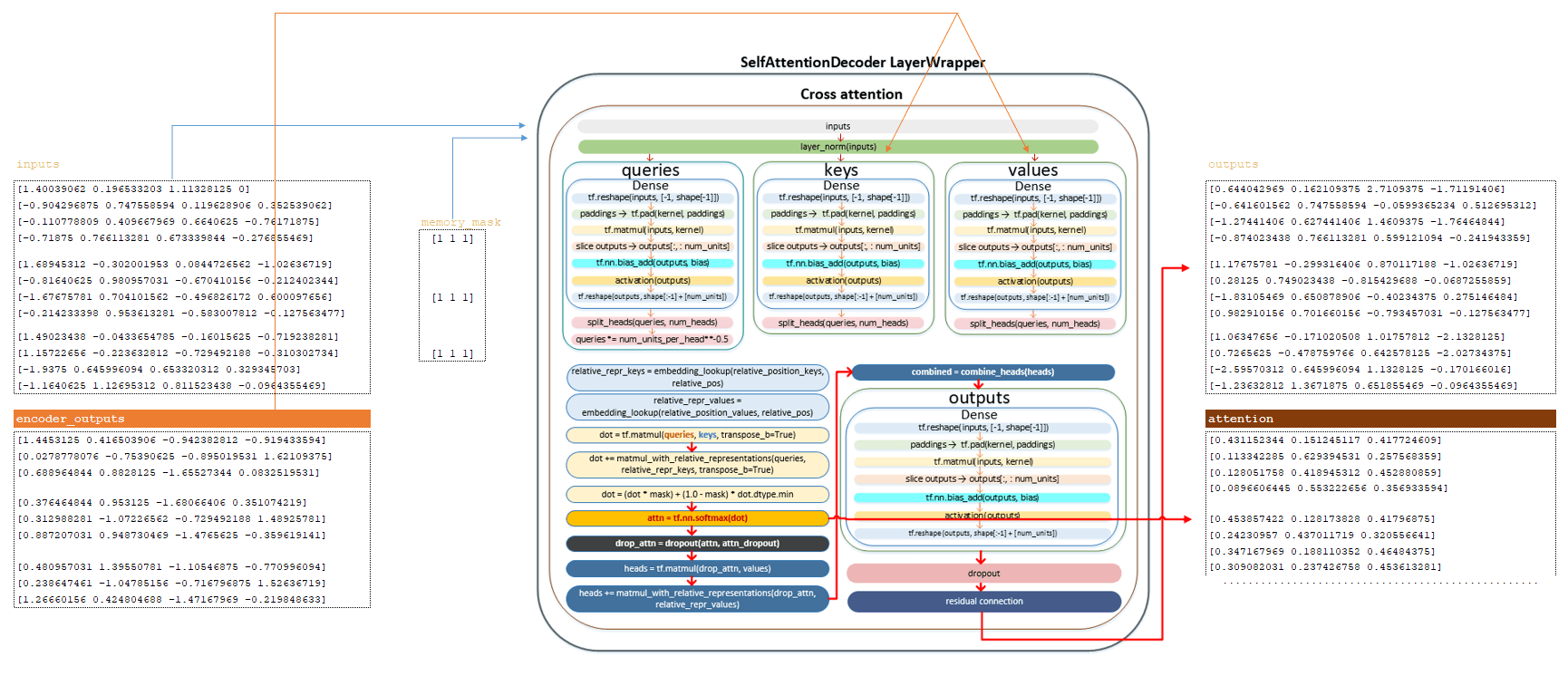
The outputs matrix is passed to the Feed Forward Network:
━ 1) normalization layer LayerNorm() is applied;
━ 2) linear transformation Dense() class. Linear transformation with ReLU activation function tf.nn.relu;
━ 3) dropout is applied (parameter ffn_dropout from the configuration file);
━ 4) linear transformation of the Dense() class;
━ 5) dropout is applied ( dropout parameter from configuration file);
━ 6) residual connection mechanism is applied.
(Image 8 - outputs_matrix)
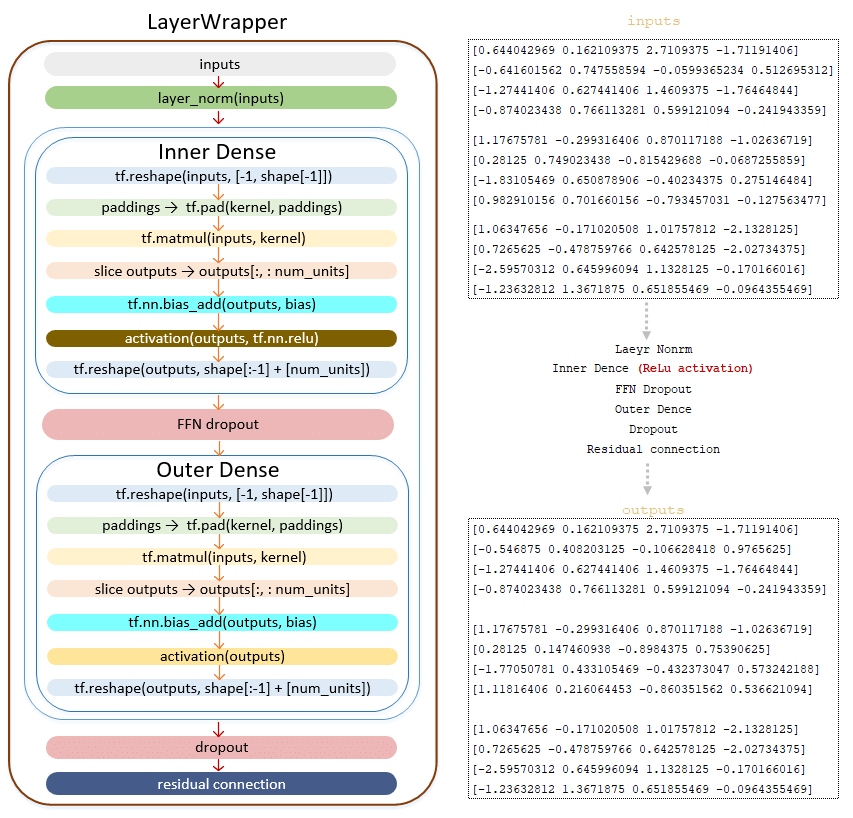
After transforming the outputs matrix using Feed Forward Network, the resulting outputs matrix passes the normalization layer LayerNorm(). (Image 9 - LayerNorm)
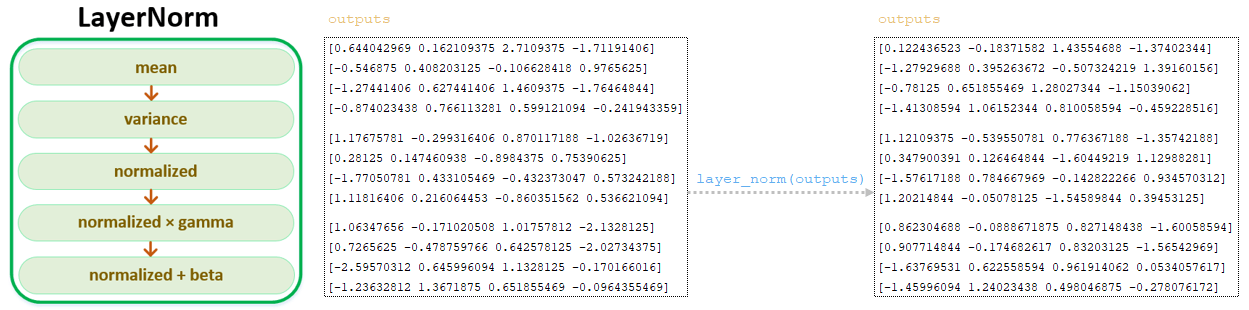
The array of attention matrices from each layer is transformed using the specified processing strategy. The default strategy is FIRST_HEAD_LAST_LAYER, i.e. the attention matrix obtained on the last layer will be taken and the first head will be taken from this matrix. (Image 10 - MultiHeadAttentionReduction )
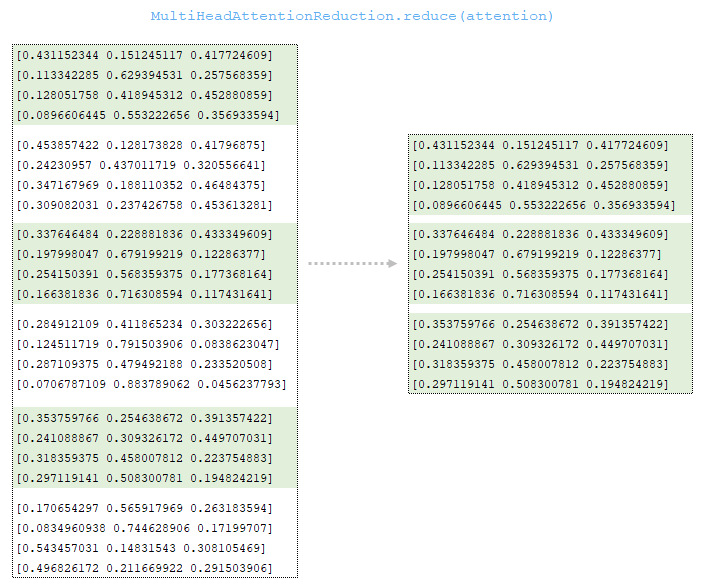
The outputs matrix after the normalization layer is transformed by the output linear layer of the decoder, whose dimension is vocab_size x num_units (in our example 26 x 4) to form the logits matrix. This transformation in the decoder is the final operation. (Image 11 - logits matrix)
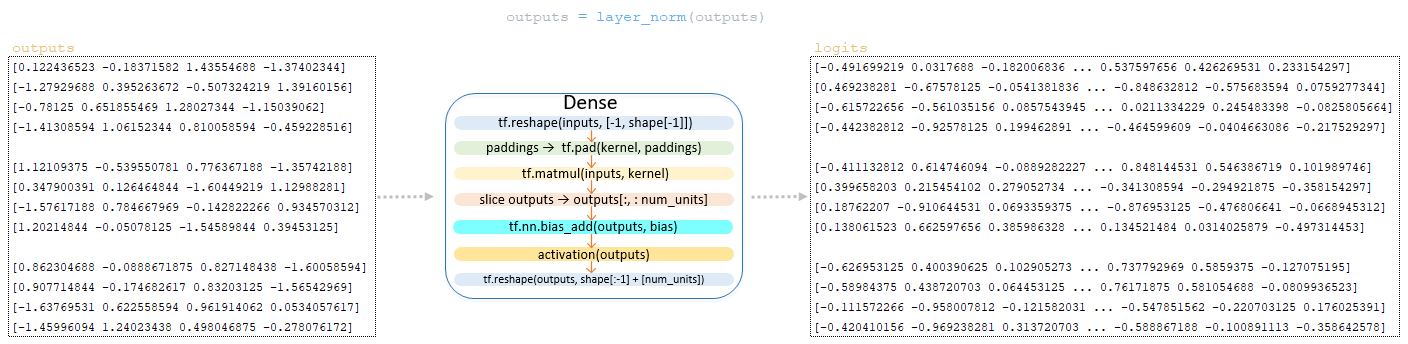
Thus, after transformations in the decoder of the target language tokens to the output of the decoder we have two matrices - the matrix of logits and the matrix of attention values from the last layer of cross attention. Schematically, the full cycle of transformations in the decoder can be represented as follows. (Image 12 - full cycle of conversions in the decoder)
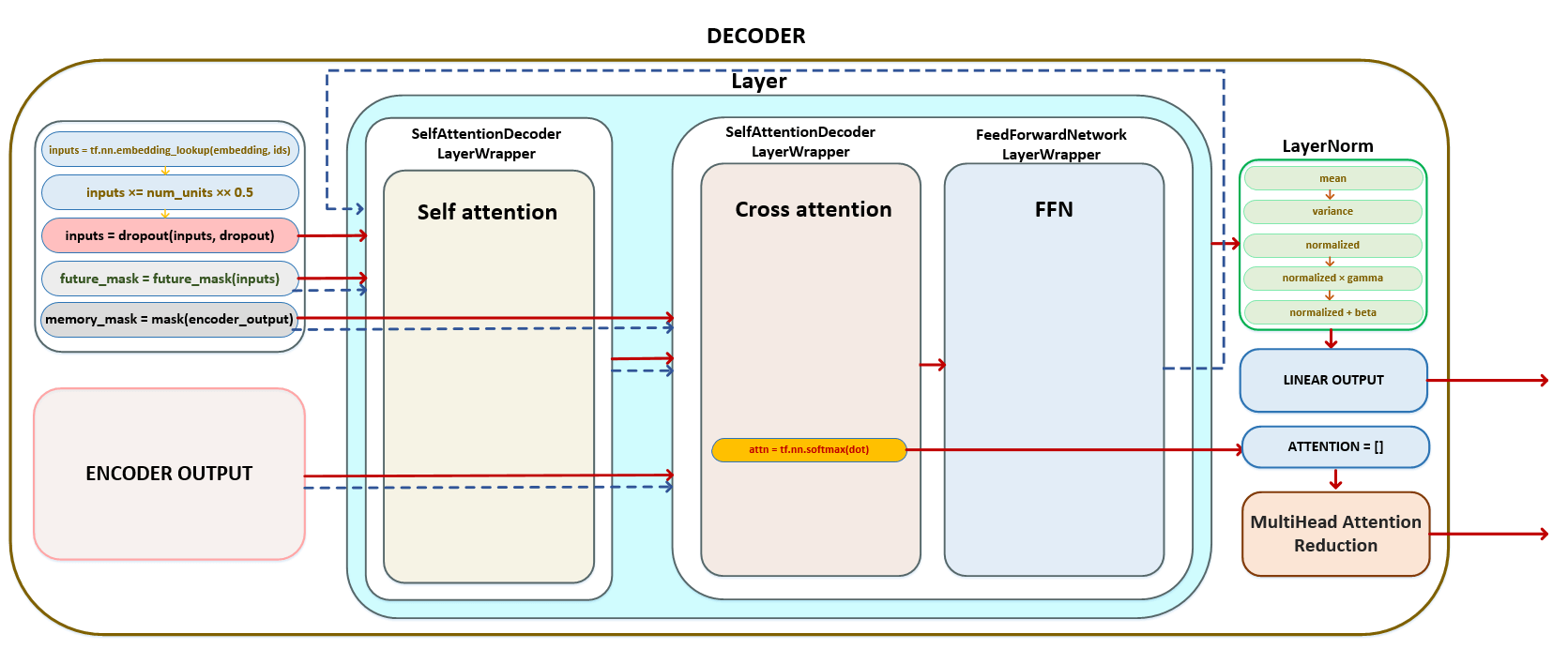
Decoder. Simplified call sequence:
├──def call() | class Model(tf.keras.layers.Layer) module model.py
├── def call() | class SequenceToSequence(model.SequenceGenerator) module sequence_to_sequence.py
├── def _decode_target() | class SequenceToSequence(model.SequenceGenerator) module sequence_to_sequence.py
├── def call() | class WordEmbedder(TextInputter) module text_inputter.py
├── def call() | class Decoder(tf.keras.layers.Layer) module decoder.py
├── def forward() | class SelfAttentionDecoder(decoder.Decoder) module self_attention_decoder.py
├── def _run() | class SelfAttentionDecoder(decoder.Decoder) module self_attention_decoder.py
├── def call() | class SelfAttentionDecoderLayer(tf.keras.layers.Layer) module layers/transformer.py
├── def call() | class LayerWrapper(tf.keras.layers.Layer) module layers /common.py
├── def call() | class MultiHeadAttention(tf.keras.layers.Layer) module layers/transformer.py
├── def call() | class Dense(tf.keras.layers.Dense) module layers /common.py
├── def call() | class LayerWrapper(tf.keras.layers.Layer) module layers /common.py
├── def call() | class MultiHeadAttention(tf.keras.layers.Layer) module layers/transformer.py
├── def call() | class Dense(tf.keras.layers.Dense) module layers /common.py
├── def call() | class LayerWrapper(tf.keras.layers.Layer) module layers /common.py
├── def call() | class FeedForwardNetwork(tf.keras.layers.Layer) module layers/transformer.py
├── def call() | class LayerNorm(tf.keras.layers.LayerNormalization) module layers/common.py
├── def reduce() | class MultiHeadAttentionReduction module layers/transformer.py
├── def call() | class Dense(tf.keras.layers.Dense) module layers /common.py
Conclusion
In conclusion, the decoder in transformer models is a vital component that effectively generates target language sequences. Through a series of carefully structured layers, it processes input embeddings, applies attention mechanisms, and produces logits for final predictions. By employing techniques like masking, normalization, and dropout, the decoder ensures robust performance in capturing contextual relationships. Understanding these processes provides valuable insights into how transformer models achieve state-of-the-art results in various natural language processing tasks.