The goal of this report is to compare translation quality between old and new language models. New models have not only improved quality but performance and memory usage. We used the BLEU metric and primarily Flores 101 test set in the report.
BLEU is the most popular metrics in the world for machine translation evaluation. Flores 101 test set was released by Facebook Research and has the biggest language pair coverage.
Quality metrics description
BLEU
BLEU is an automatic metric based on n-grams. It measures the precision of n-grams of the machine translation output compared to the reference, weighted by a brevity penalty to punish overly short translations. We use a particular implementation of BLEU, called sacreBLEU. It outputs corpus scores, not segment scores.
References
- Papineni, Kishore, S. Roukos, T. Ward and Wei-Jing Zhu. “Bleu: a Method for Automatic Evaluation of Machine Translation.” ACL (2002).
- Post, Matt. “A Call for Clarity in Reporting BLEU Scores.” WMT (2018).
COMET
COMET (Crosslingual Optimized Metric for Evaluation of Translation) is a metric for automatic evaluation of machine translation that calculates the similarity between a machine translation output and a reference translation using token or sentence embeddings. Unlike other metrics, COMET is trained on predicting different types of human judgments in the form of post-editing effort, direct assessment, or translation error analysis.
References
- COMET - https://machinetranslate.org/comet
- COMET: High-quality Machine Translation Evaluation - https://unbabel.github.io/COMET/html/index.html#comet-high-quality-machine-translation-evaluation
Lingvanex On-premise Software Updates
New version - 1.22.0.
Changes in functionality:
- Added support for audio in video files for Speech Recognizer.
New version - 1.22.1.
Changes in functionality:
- Fixed speech recognition in *.wma and *.flv files.
Improved Language Models
BLEU Metrics
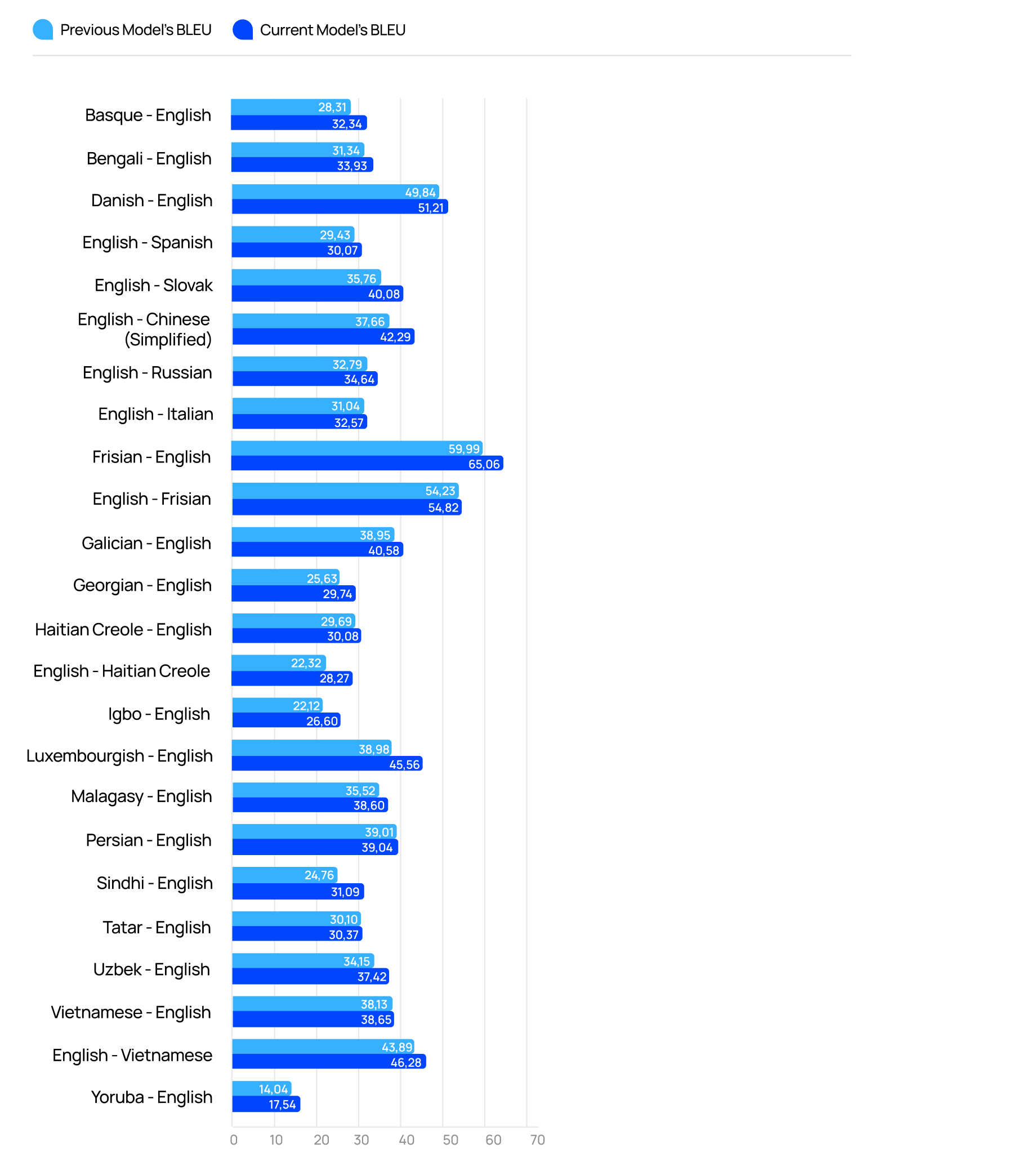
Improved Language Models. February 2024
COMET Metrics
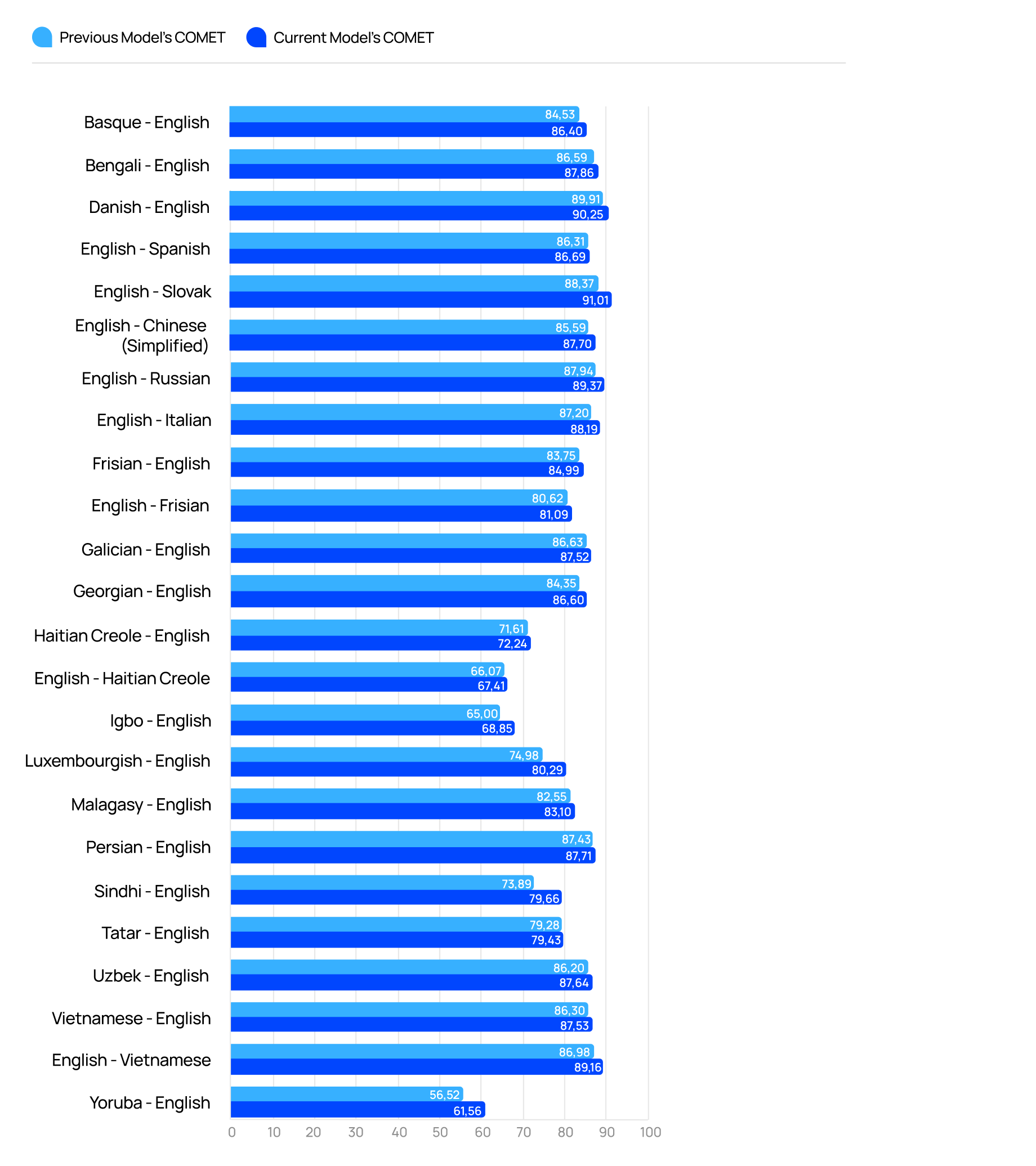
Improved Language Models. February 2024
Language pairs
Note: The lower size of models on the hard drive means the lower consumption of GPU memory which leads to decreased deployment costs. Lower model size has better performance in translation time. The approximate usage of GPU memory is calculated as hard drive model size x 1.2
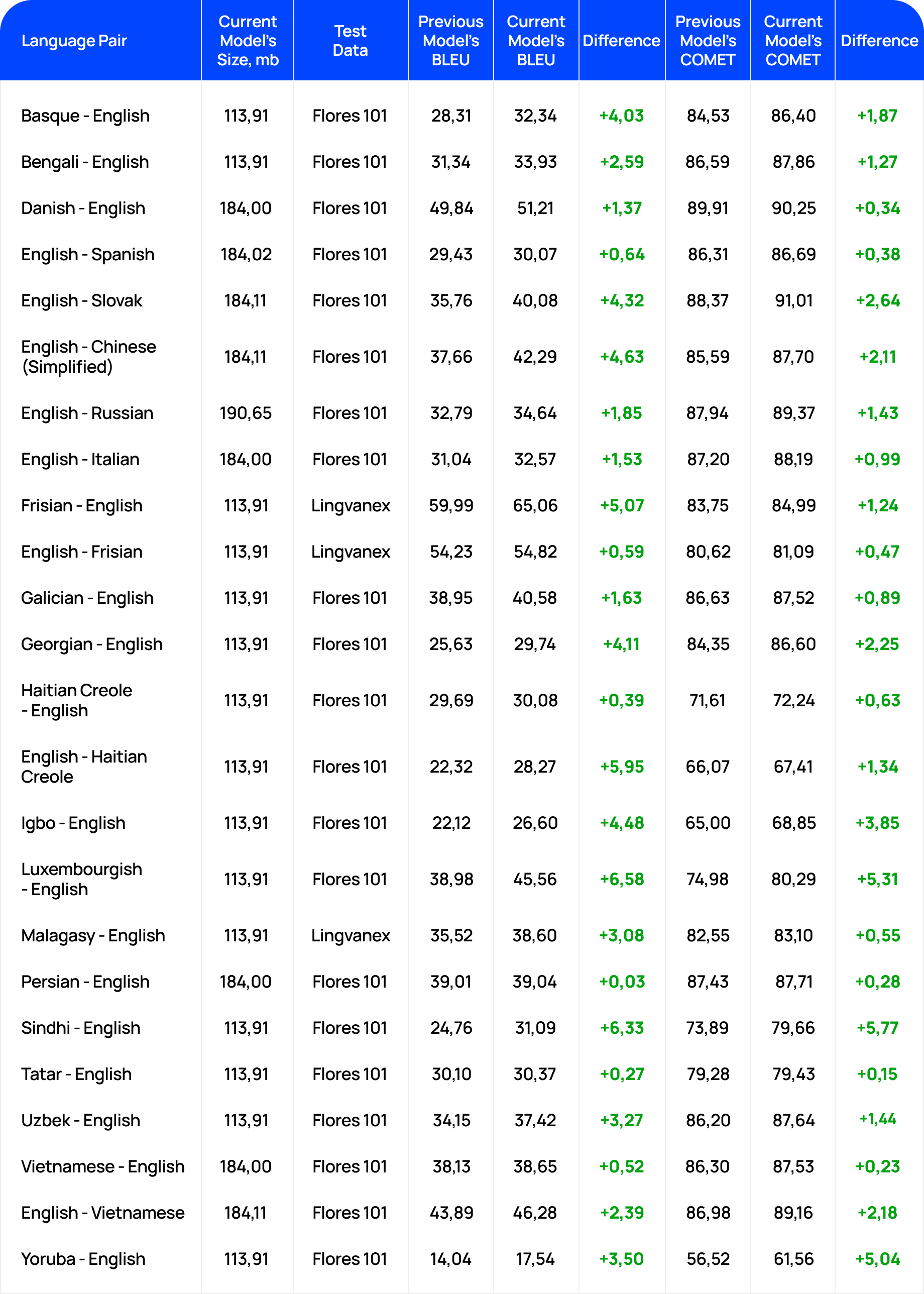
Conclusion
According to the two most popular machine translation evaluation metrics BLEU and COMET the quality of Lingvanex language models has improved significantly. The present report compares the BLEU and COMET scores of the old and new language models across various language pairs. Its major findings are that the new models have higher scores demonstrating better translation quality. The report also shows improvements in memory usage which leads to lower GPU memory consumption and helps to reduce deployment costs.