In today’s fast-paced global economy, sticking to one market or language is no longer an option for businesses. Companies strive for lightning-fast communication with customers worldwide, making neural machine translation (MT) a crucial element of any international expansion strategy. However, while translation technologies are rapidly evolving, the real challenge is not the availability of translation, but its quality and relevance to specific business needs.
Even the most advanced MT systems can fall short of meeting specific business needs. Static tests and common evaluation metrics don’t reflect real-world translation needs, especially when handling legal documents, technical specs, or culturally nuanced marketing materials.
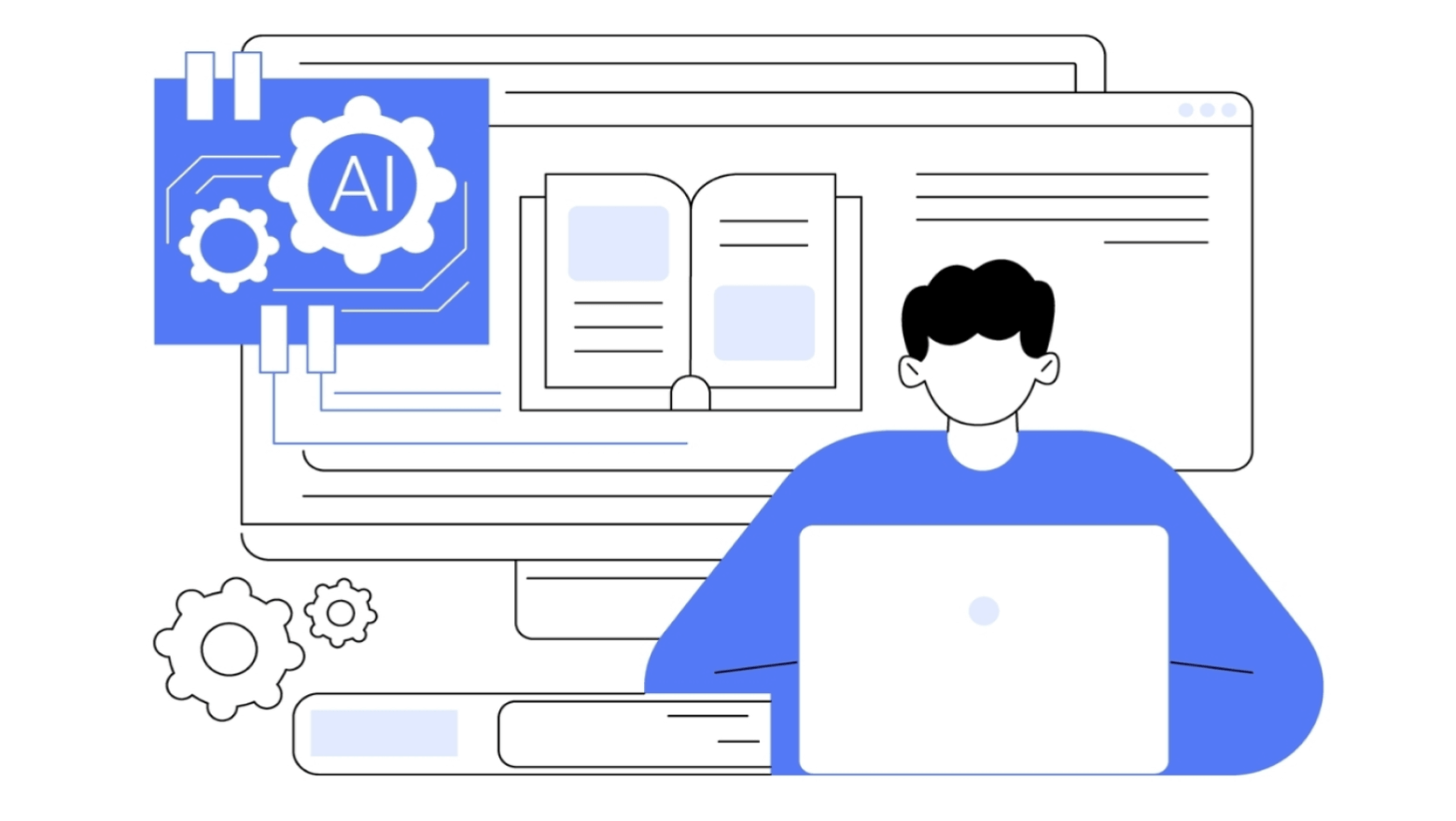
Why Accurate Evaluation of Machine Translation is Critically Important for Business
Evaluating machine translation systems is not just about comparing speed or surface-level accuracy between the original text and the translation. It's about the system's ability to adapt to the unique requirements of a business, quickly respond to changes in data, and ensure an accurate translation that maintains the meaning and stylistic features of the original text. Lingvanex offers not just translation, but an intelligent solution that adapts to your unique needs.
This article explores the technical side of MT evaluation, revealing hidden flaws in standard testing and offering innovative solutions for more practical results. We will also provide comparative testing results of Lingvanex against leading systems in the market, demonstrating how various solutions handle real-world business challenges.
Testing Machine Translation Systems: Why Standard Methods Don’t Work
Modern machine translation (MT) systems are impressive in their power and variety of capabilities, yet evaluating them remains a complex and often inaccurate task. Despite continuous technological improvements, the methods for testing and evaluating translation systems still face several challenges.
Static Test Sets: Limitations and Obsolescence
A common method for MT testing involves static datasets like FLORES or NTrex. These sets contain pre-prepared texts in various languages that translation systems must process to receive an accuracy score. However, the issue is these datasets often fail to reflect real-world usage. They often focus on narrow thematic areas or uniform sentence structures, ignoring the many nuances of natural speech and the diversity of styles that translators encounter in everyday practice.
Moreover, many test sets become outdated over time. Languages evolve, new terms, expressions, and cultural contexts emerge, which were not considered in the original data. For example, data sets created 5–10 years ago do not account for many modern linguistic and stylistic changes. Consequently, an MT system may excel in tests but underperform in real-world scenarios.
Lack of Dynamics: Context Matters
Imagine you neeв to translate a scientific article, then a piece of fiction, followed by a business letter. Each of these text types requires its own approach. But most standard testing methods don't account for changes in context and style depending on the type of content. The texts used in static sets are generally uniform and don't test how well a system adapts to different genres and styles. This leads to situations where translation systems perform well on what they were tested on but may "break" when used in real-life scenarios.
Metrics: A High BLEU Score Doesn’t Guarantee Success
Several popular metrics assess translation quality, with BLEU being one of the most well-known. This metric compares machine translations to reference translations, evaluating their similarity. However, there's a major caveat: BLEU relies on a single "correct" translation. In real life, translations can be diverse, and it’s entirely possible that multiple translations of the same text will be equally valid but different in form. BLEU doesn’t always reflect this diversity.
Moreover, BLEU and other automated metrics often don't account for the style and quality of translation in terms of readability and naturalness. A system might score high on BLEU but still produce a translation that sounds unnatural or robotic.
Data Leakage: The Déjà Vu Effect in Translation
Another issue with typical testing methods is data leakage. Some MT systems are trained on the same data used for testing. This creates a false sense of success: the system is simply "recalling" phrases it already knows and translating them correctly, without demonstrating real skills in processing new texts.
This effect can be compared to a student knowing the exam questions in advance. The results will be impressive, but it won’t reflect the true level of knowledge. In MT, this is particularly dangerous: a system may show high results on tests but fail in real tasks when it needs to translate unfamiliar content.
Data leakage happens for several reasons. First, many public data corpora used for training and testing MT systems contain overlapping fragments. This is especially noticeable when widely used data sets, such as Wikipedia or news site texts, are used. The system "remembers" some elements of the tests and produces “familiar” translation fragments, creating an illusion of accuracy. Solving this problem requires strict control over the selection of test data sets.
Solutions: New Approaches to Evaluation
The problem with typical testing methods is their static nature and uniformity. Modern MT systems need more dynamic evaluation methods that account for the diversity of context, style, and tasks users face. For example, using real texts from different fields—ranging from technical documentation to literary works—will more accurately assess a translation system’s performance in various conditions.
New metrics are also needed that evaluate not only the accuracy of the translation but also its naturalness, style, and ease of perception for humans. Evaluation should be based not only on mathematical indicators but also on human impressions and experience.
Modern companies like Lingvanex are already taking steps in this direction, developing testing systems focused on real-world usage scenarios and providing more accurate performance assessments.
Methodology for MT System Performance Evaluation: Cutting-Edge Approaches
Lingvanex offers more accurate and modern methods for evaluating machine translation (MT), aimed at overcoming the limitations of traditional tests and metrics. At the core of our methodology is the principle of adaptability and the use of real-world data, allowing for a high level of translation accuracy and naturalness. To achieve this, we use several key approaches:
- Testing on Real Data: Unlike traditional approaches that use open data sets, which can lead to information leakage or reflect common language patterns unrelated to business content, Lingvanex tests translation systems on real texts from various industries. This helps model conditions close to those our clients face, whether it be technical manuals, legal documents, or marketing materials. We analyze results in the context of specific tasks, giving a more accurate picture of how the MT system meets the real needs of businesses.
- Adaptation to Style and Context: Every type of text requires its own approach, and Lingvanex considers this when evaluating translations. We offer a system capable of adapting to different styles—from business to artistic—which significantly improves the quality of the final product. During testing, we assess how well the system handles changes in genres and styles to ensure its flexibility and ability to maintain the unique characteristics of the text.
- Multilevel Evaluation: Lingvanex uses a multilevel evaluation methodology combining automated metrics and expert assessments. In addition to the BLEU metric, we use COMET, which focuses on preserving meaning and style. For your business, this means the translation will not just be accurate but semantically correct. In marketing materials, this means maintaining emotional impact and cultural nuances. In legal texts—accurately conveying legal concepts. In technical documentation—consistency in terminology and proper use of specialized terms.
In Lingvanex, we understand that numbers don’t always tell the whole truth. That’s why we combine automated metrics with expert assessment. Our specialists conduct detailed analyses of translated texts, evaluating their quality from the standpoint of linguistic correctness, style, and audience perception.
- Data Control and Preventing Leakage: Lingvanex places special emphasis on preventing data leakage during training and testing. We develop our own test sets that do not overlap with training data and use methods that eliminate the possibility of "memorizing" phrases and expressions. This ensures the system demonstrates its true capabilities in adapting and processing new texts.
- Using Corporate Data for Testing: One of the main advantages of MT systems like Lingvanex is the ability to test them on real corporate data. These are not just publicly available data sets, but texts businesses work with daily—legal documents, technical specifications, marketing materials. Such texts often require not just translation but an accurate understanding of terminology, style, and context. Static MT systems typically perform worse on such tasks, as they cannot account for client-specific data without additional tuning. Lingvanex offers companies the opportunity to use their own data to test the system, allowing for a more accurate assessment of how the system will handle real tasks that companies face daily. This gives businesses confidence that the selected translation system will work effectively with their texts.
These advanced approaches provide a more accurate and reliable assessment of MT system performance, enabling Lingvanex to offer clients solutions that are maximally adapted to their unique requirements and real-world tasks.
Lingvanex: Adaptive Solutions for Your Business
In today’s world, static solutions can’t keep pace with rapidly changing realities, especially in machine translation. Languages are constantly evolving: new terms, technologies, and cultural changes emerge. Static MT systems cannot quickly adapt to these changes, leading to inaccurate translations or outdated terminology.
Lingvanex offers an innovative solution to this problem through an adaptive MT model that learns from your data and responds instantly to changes. This means that when new terms emerge, or industry language changes, Lingvanex updates in real time.
For example, technology companies regularly face updates in terminology. With Lingvanex, there’s no need to wait for system retraining—it instantly picks up new terms and automatically applies them in translations. This significantly reduces the time to implement new data and lowers the costs of adjusting translations.
Results That Speak for Themselves: Lingvanex Testing
To provide an objective picture of Lingvanex's out-of-the-box solution performance, comparative testing was conducted with leading competitors in the market, such as Google Translate, DeepL, Yandex Translate, GPT-4, and Microsoft Translator.
Testing was performed on real data for several languages: Spanish, Portuguese, French, German, Arabic, Hindi.
The evaluation and research data are in the public domain.
BLEU score comparison:
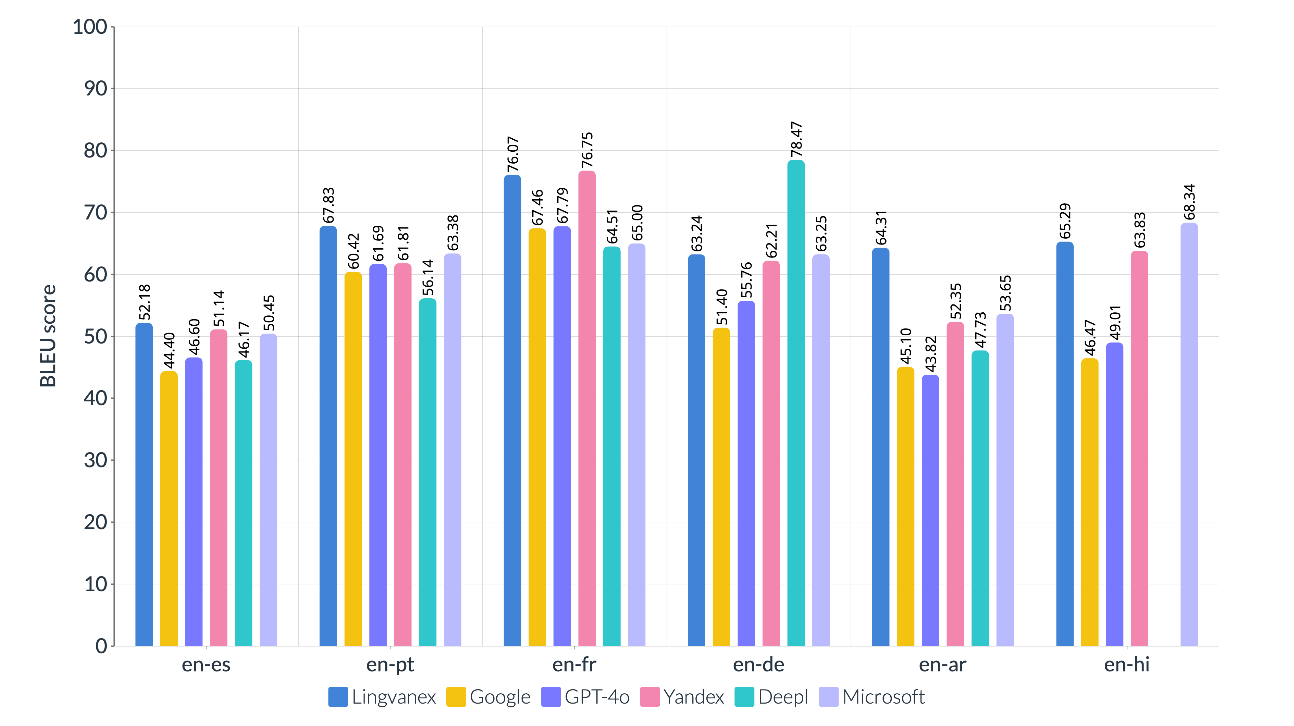
COMET score comparison:
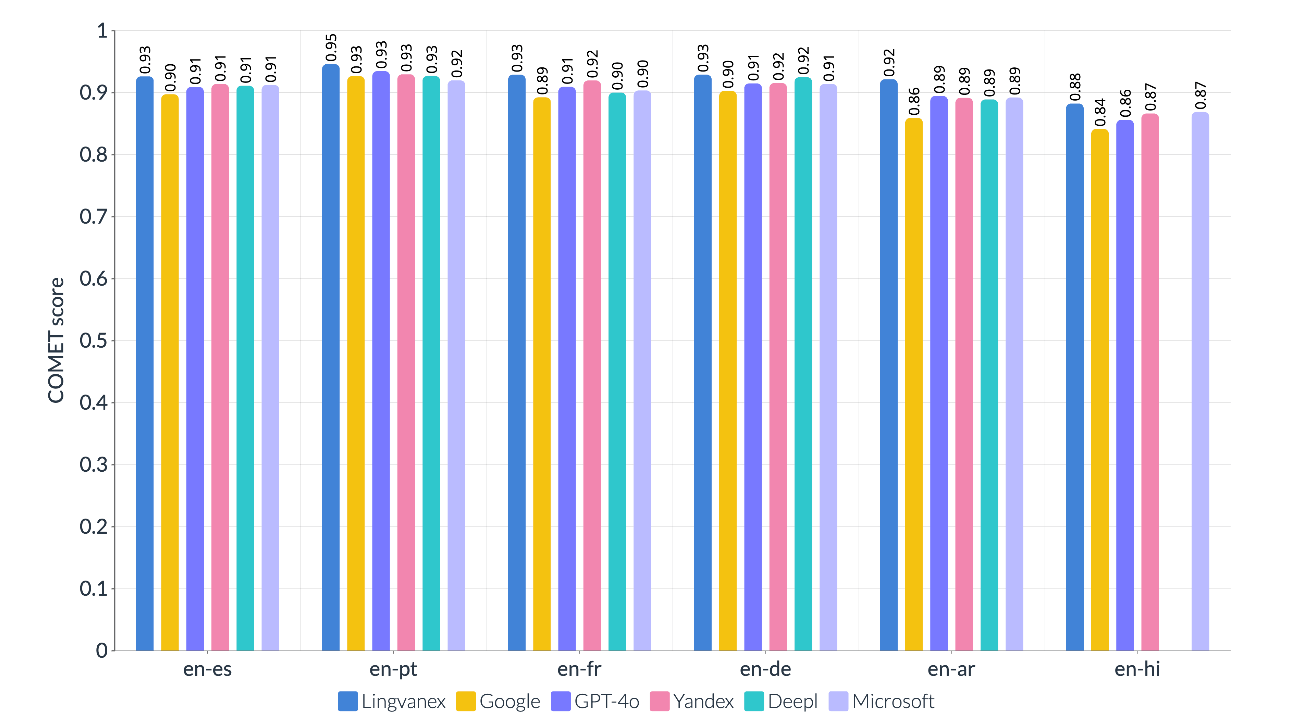
- Lingvanex is a clear leader both in BLEU and COMET scores. This indicates its ability to ensure not only accuracy at a surface level (as BLEU reflects) but also high alignment with human expectations of translation quality (COMET).
- Lingvanex excels particularly in translations into European languages, such as French, Portuguese, and Spanish, where it consistently demonstrates top results on both metrics.
- For complex languages like Arabic and Hindi, Lingvanex also maintains its leadership, although the results are somewhat lower, reflecting the challenges of translating into languages with fundamentally different structures compared to English.
The diagram reflects the test results when using Lingvanex's out-of-the-box solution. Even at this stage, the system demonstrates high levels of translation accuracy and text processing, making it effective for addressing a wide range of tasks. However, Lingvanex offers clients a unique opportunity – free customization to meet specific business needs and requirements. This can include adaptation to various domains, such as medical, legal, or financial, significantly enhancing the quality and accuracy of translations for specialized industries.
With such customization, the Lingvanex system can further improve performance by adapting to the client’s stylistic, terminological, and lexical preferences. This personalized approach allows for increased translation accuracy and better reception of the final text, making Lingvanex an indispensable tool for companies operating in specialized fields.
Conclusion: Lingvanex — Your Partner in Global Expansion
Machine translation technologies are rapidly evolving, but selecting a system that can truly meet the business’s needs is not simply about choosing the most popular platform. Standard metrics and generalized tests often fail to provide a complete picture of how a system will perform in real-world conditions. In real business, the key factor is the system’s ability to quickly adapt to a company’s unique requirements, whether it be legal precision, technical terminology, or marketing style.
Testing Lingvanex on real data and comparative results with other systems has shown that the adaptive Lingvanex model significantly outperforms competitors in terms of semantic accuracy and stylistic adaptation. This makes it the ideal choice for companies working with texts that require not just precise translation but also consideration of context, specific terms, and cultural nuances.
Lingvanex is designed to give you confidence in every translation. Our adaptive system provides localization, not just machine translation. You receive not only a quick solution but also a tool that improves the quality of interaction with international markets.