Introduction
The modern world is becoming more globalized, with language barriers being broken down daily thanks to the rapid development of technology and the introduction of artificial intelligence. One field that has seen significant changes in recent years is machine translation, which has transformed the way natural languages are translated.
The main reason causing this transformation is the integration of natural language processing (NLP) techniques, which have increased the ability of machines to understand and translate human language with greater precision, contextual awareness, and efficiency.
But how did this technology become such a powerful tool? Let’s try to explore in this article.
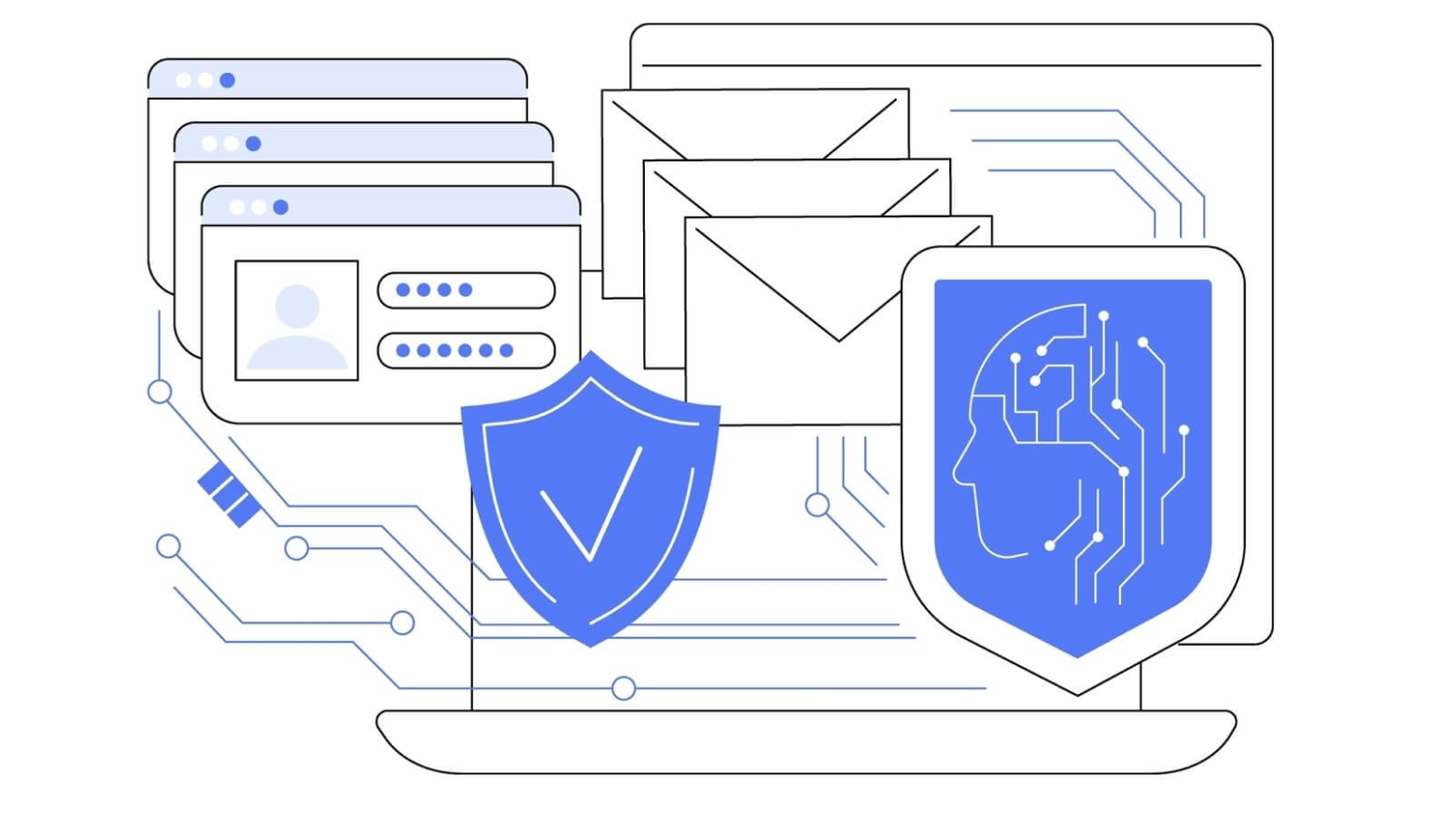
The Evolution of Machine Translation Systems
People have searched for ways to overcome language barriers and improve multilingual communication for a long time. The pursuit to achieve this has led to the resurgence of different machine translation methods.
Machine translation (MT) is the process of automatically translating text from one language to another, with the help of computer software and without direct human involvement. You can read more on this cutting-edge technology here.
Traditional machine translation systems were based on statistical methods, relying on large parallel data of translated text to build models that could predict the most likely translation for a given input. The statistical MT systems showed mediocre results: there were some limitations in capturing the nuances and complexities of a natural language.
With the invention of neural networks came the breakthrough in the field of machine translations. Neural machine translation (NMT) systems use deep learning algorithms to learn the complex patterns and relationships in a language, providing human-quality translations.
NMT systems function using an encoder-decoder framework: the encoder captures the semantic and syntactic information of the source language while the decoder generates the translated sentence, producing a more coherent and fluent output. A key feature of NMT models is the attention mechanism, which allows the system to focus on different parts of the input sentence at each step of the output generation. Due to this mechanism the neural translation models better understand the context and nuances of the language, producing more accurate and contextually relevant translations.
One more powerful force behind the rapid advancements in machine translation quality is the integration of natural language processing (NLP) techniques.
Natural Language Processing Techniques in Machine Translation
Natural Language Processing (NLP) is a field of artificial intelligence which concentrates precisely on how computers learn to recognize, analyze, and generate human speech. It is a very complex field where linguistics, mathematics, and AI overlap. The ultimate goal is to make the interaction between people and machines more natural and understandable.
Thanks to NLP, voice assistants can carry out our instructions, AI translators can translate phone calls, and chatbots can engage in meaningful dialogues. Advancements in NLP have also enabled the analysis of emotions in a text. By utilizing advanced NLP methods, modern machine translation systems can better understand the semantic and syntactic structure of the input text, and generate more coherent and fluent translations.
Incorporating NLP into the machine translation systems has enhanced its capabilities, but despite the progress achieved in translation quality during the last decade, NLP systems still face a number of limitations.
Challenges of Natural Language Processing
The limitations, still need to be addressed by natural language processing, include:
- Handling Low-Resource Languages:
Many languages around the world have limited available data, making it difficult to train effective MT models. Techniques like transfer learning (applying the data gained from high-resource languages to low-resource ones) and data augmentation (paraphrasing, synonym replacement, rule-based text generation) are being explored to address this issue. - Maintaining Contextual Accuracy:
Ensuring that the translated text accurately conveys the intended message and nuance of the original language is a constant challenge. Incorporating more advanced language understanding and generation models can help improve contextual accuracy. - Handling Ambiguity and Idioms:
Natural languages often contain lots of ambiguous expressions and idiomatic phrases that are challenging for machine translation systems to translate correctly. Continued research in areas like language understanding can help address these challenges. - Achieving Human-Level Quality:
Modern NMT still struggles to match the fluency and naturalness of human translations, especially for more complex and creative language usage. Sometimes more linguistic knowledge and human feedback are required.
All these aspects remind us that NLP-based machine translation is still a developing field. There’s a great need for continued research, innovation, and a multidisciplinary approach to address the complex challenges that lie ahead of it. The NLP developers are tasked with creating more sophisticated, inclusive, and impactful machine translation solutions.
Lingvanex is a Machine Translation Tool with NLP
One of the most notable achievements in the field of NLP is the resurgence of powerful machine translation solutions. Lingvanex's machine translation software is based on the latest advancements in NLP to provide its users with high-quality translations of websites, phone calls, messages, and documents. Lingvanex's translation engine is powered by deep learning models trained on massive multilingual datasets. This allows the tool to capture context, understand nuance, and produce translations across 109 languages that sound more natural and human-like compared to traditional rule-based translation approaches.
Conclusion
Machine translation has come a long way, transforming the way people from different countries. The integration of neural networks and advanced natural language processing techniques has enabled more accurate, contextual, and efficient translations. Natural Language Processing (NLP) plays a crucial role in the evolution of machine translation, giving access to a variety of applications that significantly simplify people’s daily lives.
However, there is still room for improvement, and ongoing research in this field promises to further enhance the capabilities of the MT technology, ultimately making global communication and collaboration more accessible.