Translation no longer relies on costly, time-consuming human efforts. With machine translation (MT) systems now offering instant solutions, the question isn't just about speed but about choosing the system that best fits your business’s unique needs.
Every company expanding into new markets understands that translating text is one thing, but doing it effectively is quite another. When selecting an MT system, it's essential to consider not only speed and convenience but also the ability to adapt to the content’s specific characteristics. This is where the real race begins between static systems and adaptive solutions.
Lingvanex, one of the rapidly evolving machine translation systems, offers more than just automated processes. It provides an adaptive model that adjusts to business content in real time.
In this article, we’ll explore how MT system performance is compared and why adaptive solutions are becoming the preferred choice for modern companies.
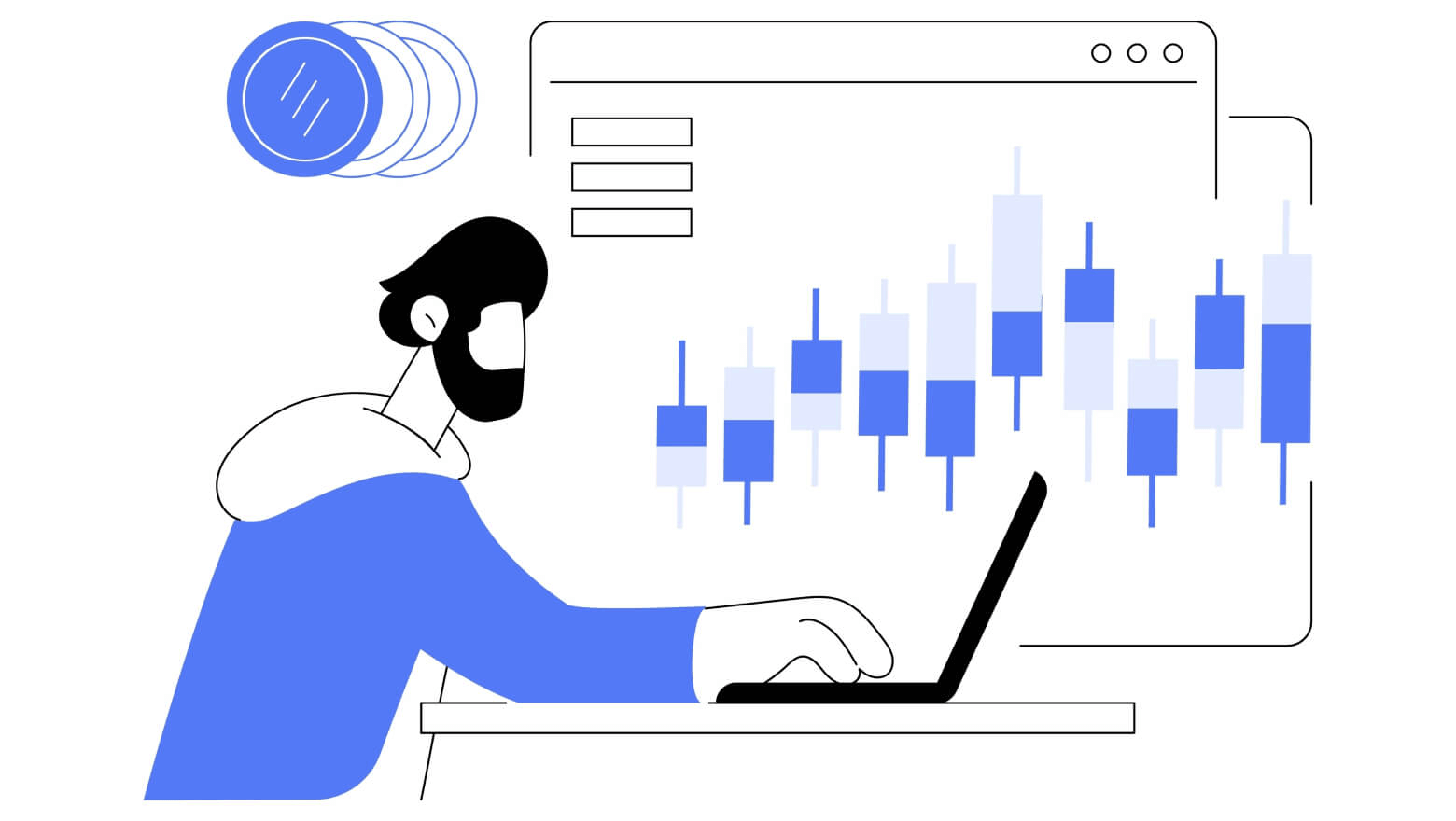
Why Comparing MT Systems Is Crucial for Businesses
The business world is changing rapidly: borders are fading, and companies are no longer confined to one market. Entering the global arena isn't just about penetrating new markets; it's about interacting with an audience that demands linguistic and cultural understanding. Automatic translation becomes a key element of this process, helping companies scale and maintain connections with customers and partners.
The global demand for machine translation has surged. According to Grand View Research, in 2023, the market reached a value of $196.63 billion and is expected to grow by 36.6%% annually until 2030. This reflects businesses’ real need for instant translation into dozens of languages. But not every MT system can meet this demand. For legal, marketing, and technical texts, it's not only crucial to ensure accuracy but also to deeply understand meaning and context.
Businesses' primary needs revolve around an MT system's ability to handle their specific content. For large tech companies launching products in 15 markets, both translation speed and accuracy are critical. Solutions like Lingvanex adapt to new terminologies, cultural nuances, and industry specifics, which is crucial for successful product localization. Hence, choosing the right system is not just a technical decision but a strategic step toward global success.
Static vs Adaptive MT Systems
In the machine translation market, companies encounter two types of systems: static and adaptive. Each offers different approaches to text processing, and understanding their features helps businesses select the optimal solution.
- Static MT systems are models based on fixed rules or pre-trained data, which are rarely updated. While they can be effective for basic needs, they have significant limitations. First, they struggle with new terms and language changes, posing problems for companies in fast-paced industries like technology, finance, or fashion. A static system might not recognize newly coined technical terms or jargon, translating them literally and distorting the meaning. Second, setting up such systems requires substantial upfront investments, including lengthy and costly data preparation and training processes.
- Adaptive MT systems, like Lingvanex, offer advantages due to their ability to "learn" in real-time. They use user feedback to continually update algorithms, adapting to new terms and language changes. This allows quick adaptation to new demands, delivering high-quality translations without delays. During the 2020 pandemic, such systems were able to quickly and accurately adjust to new realities, which was especially important for companies with dynamic content. As a result, adaptive systems reduce implementation time and offer long-term solutions, ensuring translation relevance in international markets.
Testing Performance: Methodology
When choosing an MT system, it’s not enough to rely on advertised capabilities; thorough performance testing is essential for an objective evaluation. In this section, we’ll explore testing methods and the data and metrics used to assess translation quality.
Selecting Test Data
A key element of testing MT system performance is the selection of test datasets. Widely known standardised datasets like FLORES and NTrex are commonly used in the industry for multilingual testing and include texts from various fields. However, using only these standards doesn’t always reflect real business challenges, as companies deal with translations that go beyond typical texts. Real-world texts from different domains—technical, legal, literary—are essential for comprehensive testing.
Approaches to Testing
MT system testing should cover a wide range of text types. Technical texts require term accuracy, legal documents need to be formalised, and literary texts should convey style and emotional undertones. For example, translating a user manual requires consistent terminology, while translating a novel must account for the author’s style and the target audience’s cultural context.
One effective testing method is using real user data. Companies can provide their own texts, allowing evaluation of how well the system handles industry-specific content. This is particularly useful for adaptive systems like Lingvanex, which can learn from real data and better adapt to the client’s tasks.
Key Metrics for Evaluating MT Systems
Evaluating machine translation quality is a complex task. Automated metrics provide quick and objective results, each focusing on different aspects of quality.
- COMET is one of the newest metrics aimed at assessing the semantic quality of translation. Unlike earlier methods, COMET analyzes not just the accuracy of matches between the source and translated text but also the depth of meaning. This is especially important for marketing and literary texts. However, it depends on pre-trained models and may struggle with rare languages or specific industry terms.
- BLEU is one of the most well-known metrics, simple to calculate, but it ignores semantics and word order, which can lead to high scores despite poor translation quality. SacreBLEU, an improved version, somewhat corrects this by standardizing and fixing some errors of the original version, but problems with ignoring meaning persist.
- TER measures the number of changes needed to align machine translation with a reference translation, such as word additions, deletions, replacements, or reordering. This approach is particularly useful for technical or legal documents, where precision and minimal deviation from the original are essential. However, for creative or marketing translations, where creativity is important, it may be less suitable.
Common Mistakes When Comparing MT Systems
When companies search for a suitable machine translation system, their choice often relies on the results of publicly available tests or comparisons. However, behind seemingly attractive figures and metrics, there can be errors and misconceptions that don't fully reflect how the system will perform with real business tasks. Let’s look at some of the most common mistakes.
- Testing on General Data
- The Problem of Static Comparisons
- Data Leakage and Distorted Results
- Limitations of Metrics
One common mistake is relying on results from tests with general data. These tests provide an overall evaluation but don’t reflect real business needs. A system that excels with standard texts may fail when translating specialized content. For example, in biotechnology, a system that performs well with general data may struggle to translate scientific documentation, where precision and consistency of terms are paramount.
Another frequent mistake is using a static approach when comparing machine translation systems. Many tests evaluate a system’s performance using fixed, pre-prepared data and fail to account for a system’s ability to adapt to new conditions. This creates a distorted picture, especially when comparing static systems with adaptive solutions like Lingvanex.
Adaptive MT systems are capable of "learning" and improving translation quality based on feedback and new data. When an adaptive system is evaluated in static conditions, its potential remains untapped. It's like comparing two cars in a speed test without considering that one of them can adapt to road conditions, improving its performance on challenging terrain.
A less obvious but crucial issue in evaluating MT systems is data leakage. This issue arises when a machine translation system is tested on data it has already seen during training. In other words, the model can “memorise” the test data and produce the correct answers. As a result, the system shows impressive test results, but this does not reflect its real capabilities when working with new, unfamiliar texts. Lingvanex actively combats data leakage by using strict evaluation methodologies that prevent testing on data previously used for training. You can learn more about Lingvanex's approach to selecting training and test data.
While automated metrics like COMET, SacreBLEU, and TER are important tools for assessing MT system performance, they cannot fully replace expert human evaluations, especially in specialized fields. These metrics evaluate syntax and word matches but don’t always consider the style, meaning, and emotions of the text. For example, even a minor error in a legal document can lead to serious consequences. In marketing translations, it’s not just about conveying words but also evoking the right emotions in the audience.
SacreBLEU might yield a high score for syntactic accuracy, but it could miss a mistake in translating a medical term, potentially leading to a misinterpretation. COMET, although better at evaluating semantic accuracy, may not always account for specialized terminology nuances. TER might show a low number of edits, but if those edits involve critical errors, the results could be insufficiently informative.
Human evaluation is crucial for corporate content, where accuracy, brand tone, and cultural expectations are all essential. For instance, a literal translation of a slogan may not evoke the desired response in another culture, despite a high score from an automatic metric. Only expert evaluation can capture all the nuances of translation and make it truly effective.
Lingvanex's Advantages in the MT Market
In today’s machine translation market, competition is growing, but it’s adaptive systems that offer the most significant advantages for businesses. Lingvanex stands out among other solutions thanks to its flexibility, its ability to adapt to specific client needs, and its ease of integration into existing business processes.
- Rapid Adaptation to New Domains and Content
One of Lingvanex's key advantages is its ability to quickly adapt to new domains and types of content. Unlike static systems that work with fixed datasets, Lingvanex learns in real-time, allowing it to “understand” new terms and specific texts.
Imagine a company launching a product in a market with newly developed terminology. A static system would require a long and complex setup process to handle the new terms, while Lingvanex would begin processing them almost immediately, adjusting to the company's unique requirements. This approach helps reduce the costs of manual editing and speeds up the entry into new markets. - Seamless Integration into Business Processes and Continuous Optimization
Lingvanex offers simple and flexible integration into existing systems and workflows. The Lingvanex API allows businesses to implement the solution into their platforms with minimal effort. This is especially important for large companies with extensive IT infrastructures, where changes must be made quickly without disrupting current processes.
Moreover, Lingvanex supports various data formats and usage scenarios. For example, companies can use Lingvanex to translate text on their websites, documents, and real-time communication applications. This versatility allows businesses to rely on one tool for a wide range of tasks without resorting to additional services. - Customization and Feedback Features
One of Lingvanex's most appealing aspects is the system's transparency and the ability to control translation processes. Users can customize the system according to their needs and provide feedback to further improve results. This approach is especially useful for companies that need to adhere to specific style or terminology requirements. Feedback from users helps the system learn from real data and improve translation quality.
Lingvanex is more than just a translation tool. It's a system that continually adapts, enhances its capabilities, and seamlessly integrates into any business process. Thanks to its flexibility, customization options, and transparency, Lingvanex is becoming a reliable partner for companies looking to expand globally and operate effectively in a multilingual environment.
Lingvanex: Adaptive Translation for Business
The future of machine translation lies in adaptive systems like Lingvanex. These solutions not only provide basic translation functions but also offer scalability, adaptation to unique tasks, and continuous improvement. In the era of globalisation, where language barriers can pose a significant obstacle to business growth, adaptive systems play a crucial role in ensuring accurate and efficient translation.
If your business is facing the challenge of entering new markets or improving multilingual communication, we invite you to explore Lingvanex's capabilities and see how our solutions can help you address your business challenges and accelerate your growth on the international stage.