In the new interconnected global, effective verbal exchange across languages is more important than ever. With global business expansion and intertwining cultures, exceptional translation offerings play a vital role in overcoming language limitations. The demand for accurate and contextually applicable translations has led to significant advances in translation creation, particularly the development of state-of-the-art (SOTA) models. These sophisticated models use artificial intelligence and machine learning to improve translation quality, making it faster and more reliable than traditional methods.
Lingvanex is one of the key players providing superior translation solutions for various industries. With proprietary models designed for accuracy and usability, we strive to provide the highest level of translation offerings to meet the growing needs of customers across the arena. Harnessing the energy of the SOTA era, our team not only improves translation quality but also enables people and agencies to communicate effectively across language barriers.
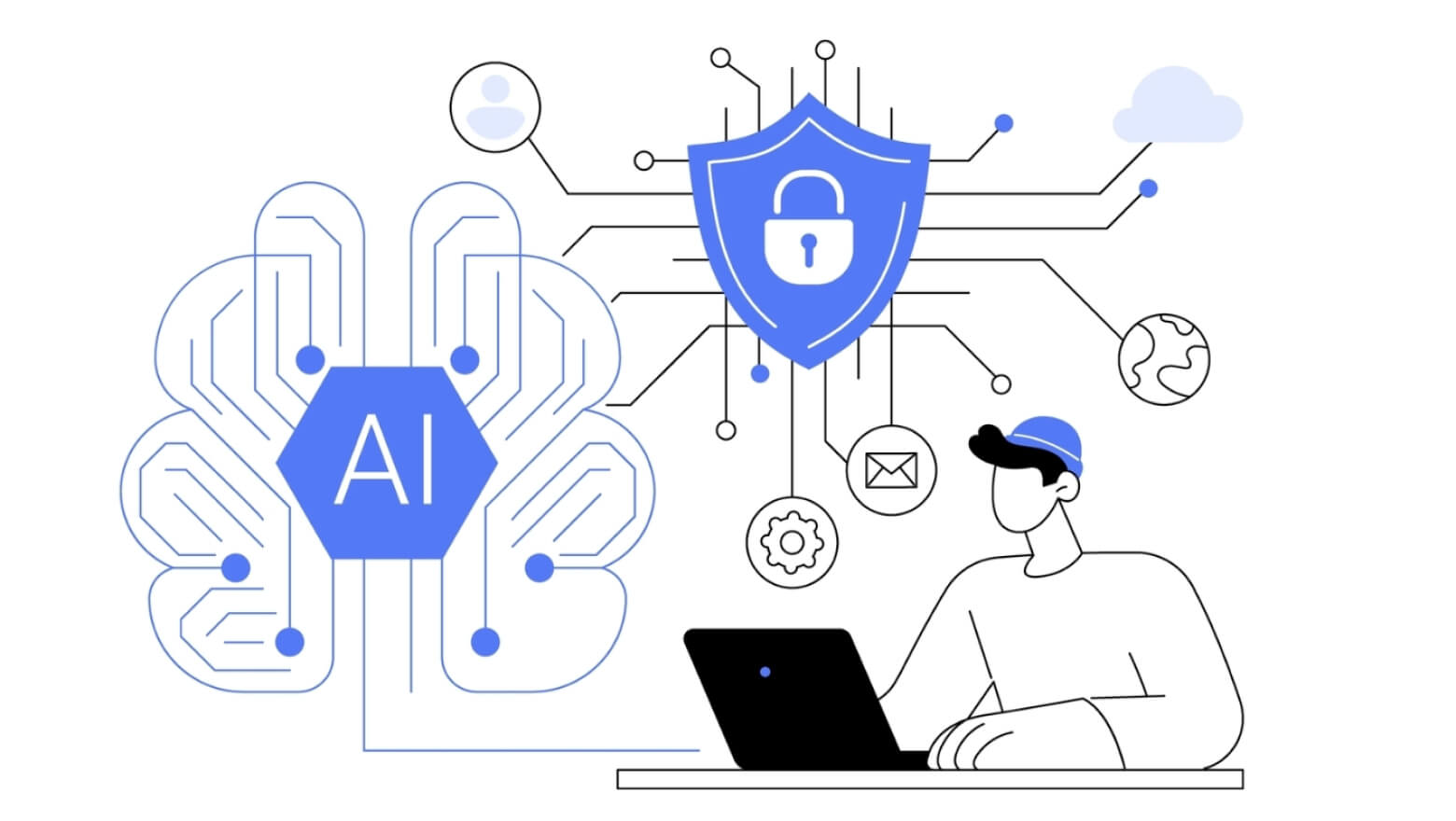
Definition of SOTA models
State-of-the-art (SOTA) models represent the height of progress in the field of machine translation, significantly improving the way languages are translated through technology. These models utilize sophisticated algorithms and neural network architectures to provide not only accurate but also contextually relevant and nuanced translation.
SOTA models in translation are generally defined as those that achieve the highest performance in standard benchmarks. They utilize deep learning techniques, particularly neural networks, to understand and generate human language. These models have changed the translation landscape by enabling computers to process and learn from vast amounts of linguistic data.
One of the most influential architect in SOTA models is the Transformer model, introduced in the paper “Attention Is All You Need” written by Vaswani et al. in 2017. Key features of the Transformer architecture include:
- Attention Mechanisms. This allows the model to focus on different parts of the input sentence while producing each word in translation, which improves contextual understanding.
- Self-attention. A special type of attention mechanism that allows the model to evaluate the importance of different words in the input text relative to each other.
- Positional encoding. Since transformers inherently do not understand word order, positional encodings are added to provide context about the position of words.
Translation models have evolved from rule-based systems to statistical models and now to neural networks. Early systems relied heavily on predefined rules and dictionaries, while statistical models used large sets of bilingual texts to generate probabilistic translations. The advent of neural networks marked a significant shift, allowing models to learn representations of words and phrases in continuous vector space, leading to smoother and more natural translations.
Recent advances in translation models
The field of machine translation has seen rapid progress in recent years, driven by innovations in artificial intelligence and deep learning. These advances have significantly improved the quality, efficiency and adaptability of translation models, making them more effective for a wide range of applications.
Notable advances in pre-trained models:
- Transformers. The advent of the Transformer architecture revolutionized machine translation. Subsequent models such as BERT (Bidirectional Encoder Representations from Transformers) and GPT (Generative Pre-trained Transformer) have built on this foundation, improving the ability to understand context and generate coherent translations. These models utilize mechanisms that allow them to better capture the relationships between words, resulting in improved accuracy.
- Multilingual models. Recently, there has been an emphasis on developing multilingual models that can handle multiple languages simultaneously. Models such as mBART (Multilingual BART) and mT5 (Multilingual T5) are designed to work well with different languages without requiring separate models for each language. This approach not only saves resources, but also improves performance in low-resource languages, improving accessibility for users worldwide.
- Fine-tuning and domain adaptation. Fine-tuning pre-trained models on specific datasets has become common practice. This allows developers to adapt SOTA models to specific domains such as law, medicine, or technical fields where specialized vocabulary and context are important. By adapting models to specific industries, the quality of translation can be significantly improved.
- Zero-shot and multi-shot learning. Modern models are increasingly capable of zero-shot or multi-shot learning, meaning that they can translate languages or dialects that they have not been explicitly trained in. This capability is especially valuable in data-poor environments or when new languages emerge, allowing for greater scope and efficiency.
Performance assessment metrics
Evaluating the performance of translation models is critical to understanding their effectiveness and ensuring high quality results. Many metrics have been developed to assess translation quality, each with its own advantages and disadvantages. This section reviews the most commonly used metrics in machine translation, providing insight into how they function and how important they are in real-world applications.
Common evaluation metrics:
- BLEU (Bilingual Evaluation Understudy). BLEU is one of the most widely used metrics for evaluating machine translation. It measures the correspondence between a machine-generated translation and one or more reference translations.It calculates accuracy by comparing the n-grams (continuous sequences of n elements) in the candidate translation and the reference translation. A brevity penalty is also taken into account to prevent translations that are too short.
- METEOR (Metric for Translation Evaluation with Explicit Ordering). METEOR accounts for some of BLEU's shortcomings by taking synonyms, stemming and paraphrasing into account.It aligns words in candidate and reference translations to calculate precision and recall. It uses a score function that evaluates matches based on word similarity and takes order and alignment into account.
- TER (Translation Editing Rate). TER measures the number of edits required to convert the system output into one of the reference translations. It counts the minimum number of edits (insertions, deletions, substitutions and transpositions) required to match the reference translation and calculates the edit ratio as a percentage of the total number of words in the reference.
- Human Evaluation. Despite the convenience of automated metrics, human evaluation remains the gold standard for assessing translation quality. Humans rate translations based on criteria such as fluency, adequacy, and overall quality. They can use scales or binary judgments to evaluate translations.
Emerging metrics:
- COMET (cross-lingual optimized metric for translation evaluation). A new metric that uses contextual embeddings from BERT-type models for translation evaluation. COMET calculates similarity scores between candidate translations and reference translations based on the learned embeddings, providing a more fine-grained evaluation.
- BLEURT (Bilingual Evaluation Understudy with Representations from Transformers). BLEURT is a metric that combines neural embeddings with traditional evaluation methods. Uses pre-trained language models to evaluate translations based on learned features, resulting in a score that more accurately reflects human judgment.
Models Used in Lingvanex
Lingvanex’s system translation software program is based on the modern improvements in NLP to provide its users with fantastic translations of web sites, smartphone calls, messages, and files. Lingvanex's translation engine is powered with the aid of deep mastering models skilled on huge multilingual datasets. This lets in the device to capture context, understand nuance, and convey translations throughout 109 languages that sound greater natural and human-like.
Lingvanex utilizes the OpenNMT-tf framework for its translation models, which are based on the classical Transformer architecture (encoder + decoder). More detailed information is available on the website Index — OpenNMT-tf 2.32.0 documentation. This approach allows for high-quality translations and optimizes the training of language models.
Lingvanex's unique offers
Lingvanex has established itself as a leader in the translation industry, providing innovative and customized solutions that leverage the latest translation technologies. This section highlights Lingvanex’s unique offerings, highlighting its commitment to quality, customization, and user experience. Lingvanex offers a range of translation services powered by state-of-the-art machine learning models that are designed to deliver high accuracy and fluency across multiple languages. The company’s models are continually updated to incorporate the latest advances in natural language processing, ensuring that users benefit from cutting-edge technology.
Key Benefits of Using Lingvanex:
- Transformer architecture. Lingvanex is based on artificial intelligence and neural networks from the Transformer architecture. These networks are based on attention and position coding mechanisms. Context is taken into account during translation, as the model remembers what was at the beginning of a long sentence and does not forget this information by the end of the phrase.
- Multiple languages. Lingvanex is designed with a global perspective: it supports more than 100 languages to meet the needs of a wide variety of users. This broad language support allows users to summarize texts in their preferred language, making it an invaluable resource for companies and individuals working in multilingual environments.
- Updates and Support. We guarantee regular updates and technical support. Our technical support team is readily available and ready to assist users with any questions or issues they may encounter. We provide comprehensive documentation, tutorials, and resources to help users maximize the functionality of the tool. With our proactive approach, our customers can count on our ongoing support, allowing them to adapt to changing needs and keep the tool relevant to their operations.
- Pricing based on volume. We offer customized plans and solutions for organizations according to their needs and requirements. We work closely with our clients to develop solutions that fit their budget and usage patterns, providing flexibility and value. Whether you are a small startup or a large enterprise, we can create a plan that fits your goals and resources, ensuring you get the most out of your investment while taking full advantage of our tools.
- Continuous Improvement. Lingvanex is committed to continuous improvement of its translation services. This includes improving and updating models based on user feedback, industry trends and advances in machine learning. By actively soliciting user feedback, Lingvanex identifies areas for improvement, such as specific translation problems and idiomatic expressions. The improvement process includes a robust feedback loop that analyzes user interactions, allowing for targeted adjustments to models. This adaptive approach ensures that tools remain relevant and meet the changing needs of different industries.
- High Accuracy. At the core of Lingvanex's undertaking is the preference to provide notably accurate and linguistically fluent translations. To achieve this aim, Lingvanex utilizes superior algorithms and models trained on a number of datasets, allowing them to capture the subtleties of different languages. High accuracy is ensured via complete education that exposes the fashions to a extensive range of language structures and vocabulary, allowing them to handle complex sentences and specialised terms. Accuracy is supported by means of rigorous testing in opposition to installed benchmarks, which promotes information-driven performance improvement.
Conclusion
Language communication cannot be overstated. Quality translation services are essential to overcome language barriers, increase global business enterprise, and facilitate cultural exchange. Advances in translation technology, especially through the latest trends (SOTA), have changed the landscape of gadget translation, making it faster, more accurate, and contextually relevant.
Lingvanex is one of the key players in this field. With a commitment to continuous improvement, high accuracy, and reliable user guidance, Lingvanex now not only meets the growing needs of its customers, but also improves the overall level of communication. As translation technology continues to adapt, the ability to seamlessly communicate in two languages will only increase, allowing people and agencies to collaborate more effectively in our globalized society.