In the era of globalization and the rapid development of technology, the need for effective and accurate translation of texts is becoming increasingly urgent. Adaptive machine translation (AMP) is one of the most promising areas in the field of automated translation, which uses the capabilities of machine learning to improve the quality and speed of translation.
Traditional machine translation systems, such as statistical and neural translation, often face problems related to context, specific terminology, and language nuances. Adaptive machine translation solves these problems by allowing systems to "learn" based on user experience and specific requirements, which in turn contributes to improving translation results.
In this article, we will look at the concept of adaptive machine translation, how it differs from traditional machine translation, we will also look at the advantages and disadvantages of adaptive machine translation.
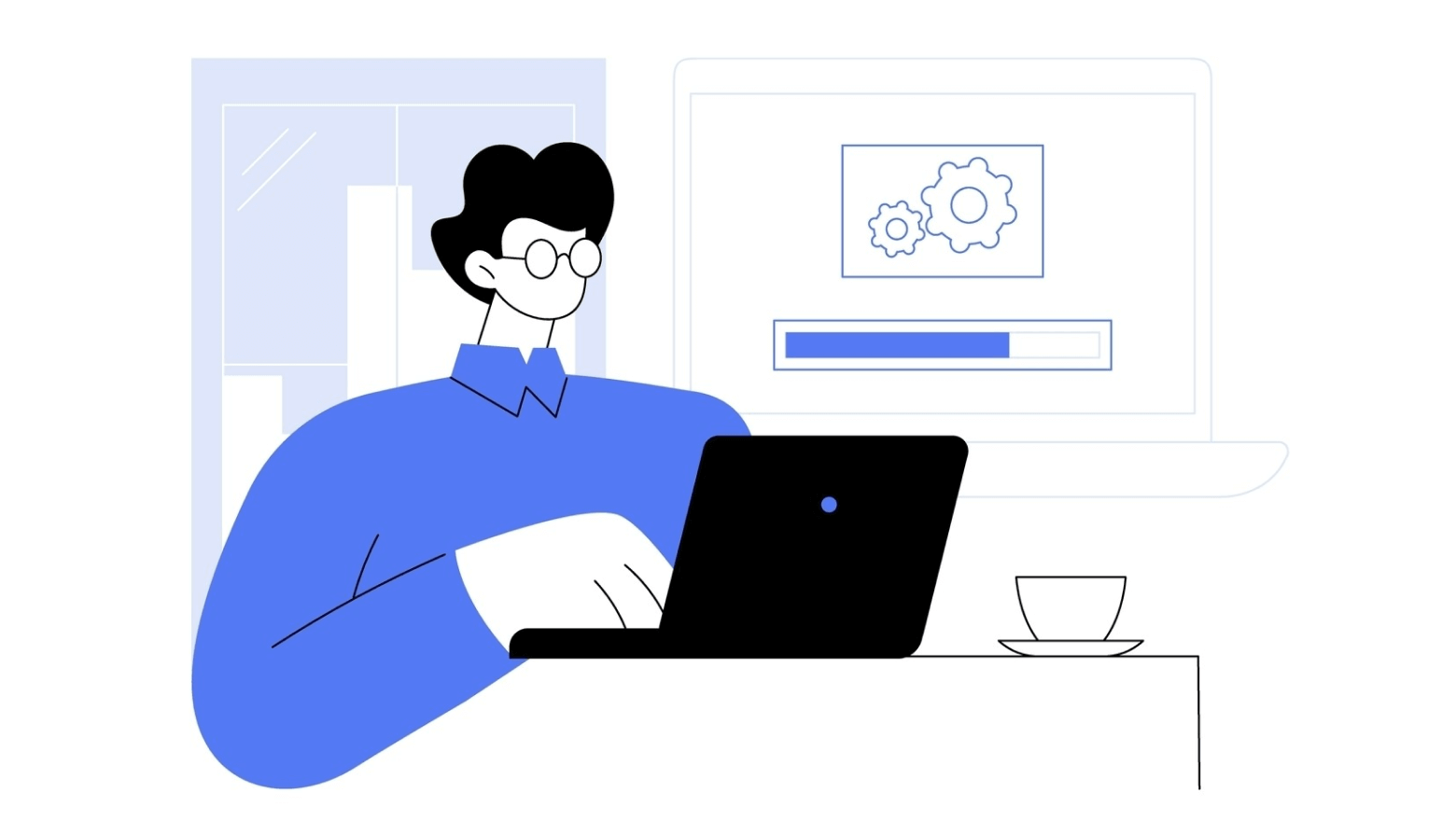
What is Adaptive Machine Translation
In adaptive machine translation, the system is customized while the human post-editor fixes the machine translation output, instead of after batch retraining. Adaptive machine translation is an example of online machine learning and human-in-the-loop (HITL).
Adaptive machine translation (AMT) is a type of machine translation system that can adjust its translation output based on user feedback, context, or specific domain requirements. Unlike traditional machine translation systems that rely on fixed algorithms and pre-trained models, adaptive systems can learn and improve over time by incorporating new data and user corrections.
In adaptive machine translation, the system is tailored in real-time as the human post-editor corrects the machine-generated translations, rather than waiting for batch retraining. This approach exemplifies online machine learning and incorporates a human-in-the-loop (HITL) methodology.
How Does Adaptive Machine Translation Work
Adaptive machine translation (MT) systems typically begin with initial training, where they utilize a large dataset of bilingual texts, known as parallel corpora, sourced from various materials such as books, websites, and previous translations. Through machine learning techniques, the system learns to translate between languages by identifying patterns within this training data.
Once deployed, user interaction becomes essential. The system collects feedback from users in the form of corrections, ratings, or preferences, which is vital for its adaptation. Additionally, it can analyze the context in which translations are used, allowing for adjustments tailored to specific domains, such as legal or medical fields, or to individual user preferences.
The process of incremental learning follows, where the system periodically updates its translation model based on the accumulated feedback and new data. This can involve retraining certain parts of the model or fine-tuning it with fresh examples. Some adaptive MT systems even enable real-time adaptation, adjusting translations immediately as users provide corrections.
Furthermore, domain-specific customization enhances the system's capabilities. It can personalize translations for specific users or industries by learning the terminology and phrasing commonly used in those contexts. Maintaining user profiles helps the system remember individual preferences and past corrections, further refining its output.
Evaluation and performance monitoring are critical components of the adaptive MT process. The system regularly assesses translation quality through metrics like BLEU scores or user satisfaction ratings, identifying areas that require further adaptation. A/B testing may also be employed to compare different translation versions and determine which performs better in real-world scenarios.
Lastly, adaptive MT systems often integrate advanced technologies such as natural language processing (NLP) to better grasp context, idiomatic expressions, and linguistic nuances. Many modern systems leverage neural networks, particularly transformer models, which excel at capturing complex language patterns, enhancing the overall translation quality.
Difference Between Adaptive MT And Static MT
Adaptive Machine Translation
Adaptive machine translation systems possess a remarkable learning capability, allowing them to evolve through user interactions and feedback. Over time, they refine their translations based on user corrections, preferences, and the specific context in which they are used. These systems are also capable of dynamic updates, meaning they can adjust their translation models in real-time, incorporating new data to enhance accuracy as they are utilized. This adaptability enables personalization, as the translations can be tailored to individual users or particular domains, making them more contextually relevant. As a result, adaptive systems generally demonstrate superior performance in specialized contexts or for specific users, continuously improving with increased usage. Advanced examples of this technology can be found in various commercial applications, where adaptive techniques are employed to enhance translation quality over time.
Static Machine Translation
Static machine translation systems operate with a fixed model that remains unchanged after deployment, meaning they do not learn from user interactions. This lack of real-time learning restricts their ability to adapt based on user feedback or new data, which can diminish their effectiveness in dynamic or specialized contexts. While static MT is generally designed to perform well across a broad range of texts, it may not excel in niche applications or meet specific user needs. Although these systems can be fast and efficient, they often struggle with translations that require an understanding of context-specific nuances. Traditional rule-based or statistical MT systems typically exemplify this approach, as they are built and deployed without any ongoing learning capabilities.
In essence, the key difference lies in adaptability and learning: Adaptive MT evolves and personalizes based on user interactions, while Static MT relies on a fixed model that does not change after deployment. Adaptive MT tends to provide more accurate and relevant translations over time, especially in specialized contexts.
Benefits Of Adaptive Machine Translation
Adaptive Machine Translation (MT) provides significant advantages over traditional machine translation systems by utilizing real-time learning from user feedback to improve translation accuracy and efficiency. One major benefit is continuous learning, where adaptive MT systems evolve based on user corrections, allowing for dynamic enhancements in translation quality with each interaction. This adaptability means the system can accommodate specific terminology and user preferences without requiring extensive retraining.
Another advantage is the ability to implement immediate updates. Unlike traditional MT systems that rely on batch training, adaptive MT allows for instant changes, resulting in quicker project initiation and enabling translators to observe the effects of their corrections in real-time, thus streamlining their workflow.
Cost efficiency is also a key benefit, as adaptive MT reduces the necessity for extensive human post-editing, leading to significant savings—organizations can cut translation costs by over 60% while still delivering high-quality results, as the system minimizes repetitive tasks.
Another important feature is context sensitivity; many adaptive MT systems provide context-aware translations that enhance relevance and accuracy by considering the surrounding text. This is especially useful in specialized fields like legal or medical translation, where maintaining consistent terminology is essential.
Moreover, translators gain enhanced control over the output, as they can directly influence the system's learning through their feedback. This facilitates easier management of terminology and ensures consistency throughout projects.
Studies indicate that adaptive MT can boost human reviewers' productivity while maintaining quality comparable to traditional systems. The dynamic nature of adaptive MT transforms the approach from static suggestions to an ever-improving model.
Scalability is another advantage, particularly for organizations requiring rapid translation of large volumes of content. The technology efficiently manages extensive datasets, making it ideal for enterprises aiming to broaden their global presence quickly.
Finally, integrating adaptive MT into workflows significantly improves the user experience for translators by alleviating frustrations linked to low-quality outputs and fostering a more collaborative environment between humans and machines. Overall, adaptive Machine Translation enhances translation quality, efficiency, and user satisfaction through continuous learning and personalization, making it an invaluable tool across various applications and industries.
Limitations of Adaptive Machine Translation
Adaptive Machine Translation (MT) offers considerable advantages, yet it encounters several challenges that can impede its effectiveness and widespread adoption. One significant issue is the scarcity of training data; these systems depend on user feedback for improvement, but often lack sufficient and relevant datasets. Static models typically require large volumes of data to achieve meaningful enhancements, and without enough corrective input, their performance may stagnate.
Additionally, user experience and usability present hurdles, as many individuals struggle with the interfaces of adaptive MT systems, leading to frustration. Problems such as broken hyperlinks, undetected spelling errors, and mishandled tags can detract from the overall user experience. Furthermore, a steep learning curve is often necessary for users to fully utilize the interactive features, which can discourage initial adoption.
Another challenge is the variability in translation quality; users frequently report issues like grammatical mistakes, unrecognized terms, and inconsistencies in terminology. These problems often stem from the system's reliance on real-time corrections that may not always be accurate or contextually suitable.
The complexity of managing multiple models also poses difficulties, as it can lead to potential misalignment errors and overwhelm teams responsible for ongoing maintenance, resulting in operational inefficiencies. Moreover, many adaptive MT systems struggle with context sensitivity, failing to maintain the necessary sentence- and document-level context essential for producing high-quality translations. Without adequate context awareness, translations can lack coherence and accuracy, especially in more complex documents.
Use Cases of Adaptive Machine Translation
Adaptive Machine Translation (AMT) refers to systems that can learn and improve from user interactions and feedback over time. One of the key applications of AMT is personalized translation, which allows for adaptations based on individual user preferences, linguistic styles, or specific terminologies relevant to their industry. This significantly enhances user satisfaction by providing more accurate and contextually relevant translations.
AMT is also widely used in specialized fields, such as legal, medical, and technical documents, where precise terminology is crucial. This improves reliability and reduces the likelihood of errors in important documents. In real-time applications, AMT can be integrated into messaging platforms and video conferencing tools, facilitating seamless multilingual communication and enhancing collaboration between speakers of different languages.
Additionally, AMT plays a vital role in content localization, which involves adapting marketing materials, websites, and software interfaces to align with the cultural and linguistic preferences of various regions. This increases engagement and effectiveness of content in diverse markets. In customer support automation, AMT can be integrated into chatbots and automated response systems, allowing for multilingual support and significantly improving the user experience.
In the educational sector, AMT helps adapt learning materials and online courses for a broader audience, enhancing accessibility and learning opportunities for non-native speakers. In social media, AMT is used for translating posts, comments, and reviews, making them understandable across different language groups and fostering a more inclusive online community.
Adaptive Machine Translation can also be utilized in voice assistants and smart devices, improving the accuracy of speech recognition and translation, which leads to more natural interactions with technology. In translation memory systems, AMT can enhance traditional approaches by learning from user inputs and corrections, increasing efficiency and consistency in translations over time.
Finally, AMT can be employed for the automatic translation of news articles and media content in real-time, ensuring that important information reaches global audiences quickly and accurately. In summary, Adaptive Machine Translation has the potential to revolutionize communication across language barriers by providing more accurate, context-aware, and personalized translations, highlighting its significance in today’s globalized world.
Lingvanex Adaptive Machine Translation
Lingvanex is a versatile adaptive machine translation (MT) system that offers several advantages, making it a strong contender in the field of language technology. Here are the key benefits of using Lingvanex:
- Multilingual Capabilities. Lingvanex supports translation in 109 languages, allowing users to reach a global audience. This extensive language coverage is beneficial for businesses operating in diverse markets.
- High-Speed Translation. The system is designed for high-speed multilingual translation, capable of processing billions of characters per day. This efficiency is crucial for organizations that require rapid translations for large volumes of content.
- Integration Flexibility. Lingvanex offers easy and quick integration into various products and platforms, making it adaptable for different business tasks. This flexibility allows companies to incorporate the translation capabilities seamlessly into their existing workflows.
- Contextual Understanding. The use of artificial intelligence and machine learning enhances Lingvanex's ability to provide accurate contextual translations. This capability helps in delivering translations that are not only linguistically correct but also contextually appropriate.
- Offline and On-Premise Solutions. Lingvanex provides both on-premise solutions and offline translation modes, ensuring data privacy and security. This feature is particularly appealing to organizations concerned about sensitive information in their translations.
- Cost-Effective Solutions. With flexible pricing options, including fixed-price translations without limits, Lingvanex offers cost-effective solutions suitable for various budgets. This approach can help organizations manage translation costs effectively.
- User-Friendly Interface. Users have reported that Lingvanex is easy to use, making it accessible for both business and personal activities. A user-friendly interface can significantly enhance user experience and encourage adoption.
- Continuous Improvement. The adaptive nature of Lingvanex means that it can learn from user interactions over time, improving its output based on corrective feedback. This continuous learning process enhances the quality of translations as the system evolves.
- High Stability Under Load. Lingvanex is noted for its stability even under heavy load conditions, ensuring reliable performance during peak usage times. This reliability is crucial for businesses that depend on consistent translation services.
Lingvanex exemplifies the capabilities of adaptive machine translation systems, offering a blend of advanced technology, user customization, and practical applications that cater to a diverse range of users and industries.
Conclusion
Adaptive machine translation is a transformative approach to language processing that enhances communication across diverse contexts. By learning from user interactions, adapting to specific needs, and incorporating contextual awareness, AMT systems are revolutionizing how we approach translation. Embracing this innovative approach not only improves the quality of translations but also fosters better communication in our interconnected world. Organizations looking to thrive in a global marketplace must consider the advantages of adaptive machine translation in their strategies.